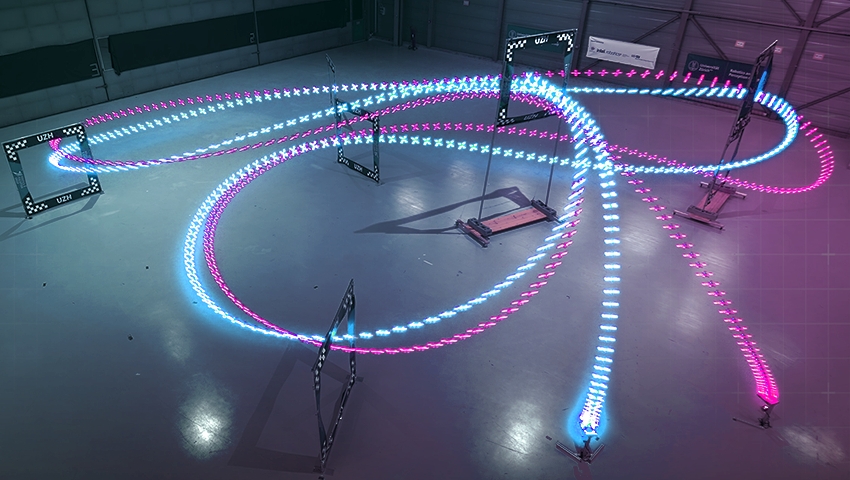
Researchers at the University of Delft University of Technology (TU Delft) use Drone racing to test AI systems based on the neural network intended for future space missions. This innovative research was a collaboration between the Advanced Concepts team from the European Space Agency (ESA) and the Micro Air Vehicle Laboratory (MAVLAB) in Tu Delft.
The project aims to explore the use of training networks to manage complex spacecraft maneuvers, such as interplanetary transfers, surface landings and dockings. In the difficult environment of space, maximize the efficiency of on -board resources, including propellant, energy, computing power and time, is crucial. Neurons networks have the potential to optimize operations on board, improving both mission autonomy and robustness.
To validate these neural networks in real world conditions, researchers have chosen drone race as an ideal test field. The Cyber ​​Zoo, a 10×10 meter test area at the Aerospace Engineering Faculty of Tu Delft, provided the perfect setting. Here, human pilot drones alternate with autonomous drones equipped with networks of neurons formed by various methods.
Drone Racing is an excellent test field for end-to-end neural architectures on real robotic platforms, helping researchers strengthen their applicability to space missions. The drones have traveled a defined course, simulating the constraints and challenges that spacecraft would meet during the missions.
Traditionally, spaceship maneuvers are meticulously planned on the ground, then downloaded from the spaceship. The guidance part occurs on earth, while the control party is managed by the spaceship. However, the unpredictable nature of space, with variables such as gravitational changes and atmospheric turbulence, poses significant challenges.
The alternative approach, called end -to -end guide and control networks (G&C NTS), implies all the processes taking place on the spacecraft. Rather than taking a predetermined course, the spacecraft constantly resumes its optimal trajectory of its current position, resulting in much greater efficiency. This method considerably reduces resource costs associated with traditional brute force corrections.
There are many similarities between drones and space vessels, although drone dynamics is faster and noisier. In the race, time is the main constraint, but it can be used as a proxy indicator of other essential variables to spatial missions, such as the mass of the propellant.
Despite the limits of satellite CPUs, the Net G&C are surprisingly compact, storing up to 30,000 parameters in memory using only a few hundred kilo-kilo-kilo-kilo-kilo-kilo-ket involving less than 360 neurons.
For G&C nets to be effective, they must send commands directly to actuators – propellants for spacecraft and propellants for drones. The main challenge was to take up the reality gap between simulated and real actuators. The team approached it by teaching the neural network to adapt to the conditions of the real world. For example, if the propellers provide less thrust than expected, the drone detects this via its accelerometers, encouraging the neural network to adjust orders to follow a new optimal path.
The dynamic academic community of drone races offers an excellent opportunity to test and refine these systems. The use of drones helps to establish a solid theoretical framework and to define security parameters before planning a real spatial mission.
Cyber ​​zoo drones are not just in speed for speed, but also paves the way for a future space exploration. By refining AI control systems based on neural networks in this demanding environment, ESA and Tu Delft make significant progress towards more autonomous and effective space missions.
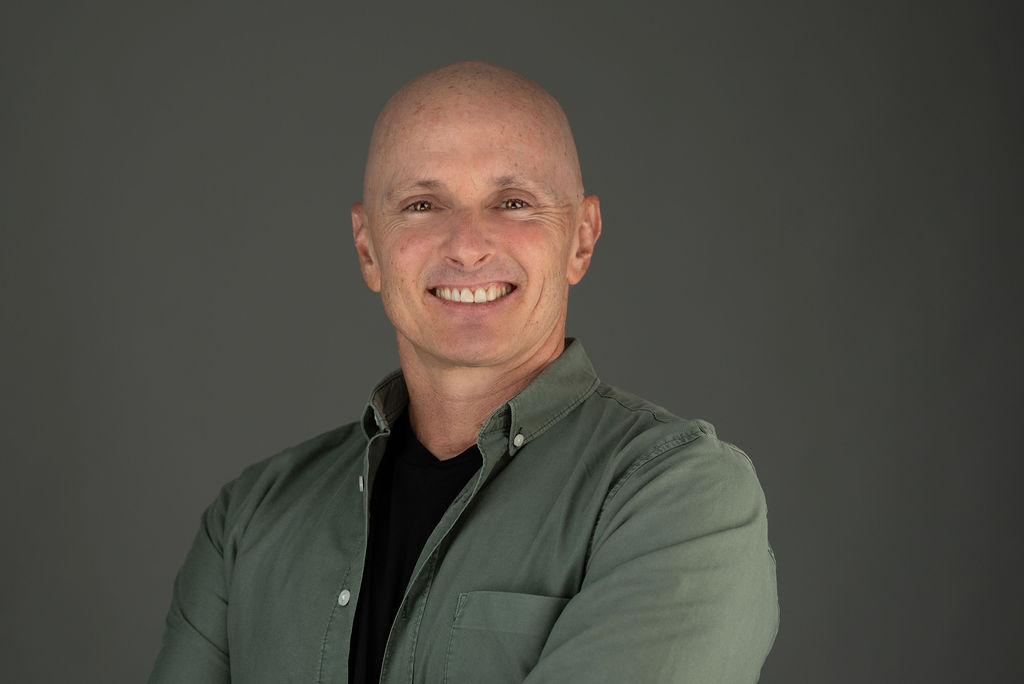