The deployment of agents based on the large language model (LLM) in production parameters often reveals critical reliability problems. It is essential to identify with precision the causes of the failures of the agents and to implement proactive self-correction mechanisms. Recent analysis of ATLA on the public bench τ Benchmark provides granular information on agents' failures, going beyond the traditional measures of global success and highlighting the Evaltoolbox approach in Atla.
Conventional evaluation practices are generally based on aggregate success rates, providing minimal feasible information on the real reliability of performance. These methods require manual journals of extended newspapers to diagnose problems – an uncapable approach as deployments are on the scale. Rely solely on success rates, such as 50%, provides insufficient clarity concerning the nature of remaining unsuccessful interactions, complicating the troubleshooting process.
To fill these evaluation gaps, ATLA has carried out a detailed τ-bench analysis-a reference specially designed to examine the tool-to-user-user interactions. This analysis systematically identified and categorized the failures of the workflows of agents in τ-retail, a subset focused on retail customer service interactions.
Explore an overview of ATLA Evaltoolbox (launch soon) hereAnd register To join the ATLA user community. If you want to know more, book a call with the ATLA team.
A detailed assessment of the key default categories highlighted τ-recentric:
- Work flow errorsMainly characterized by scenarios of “bad action”, where the agents failed to perform the necessary tasks.
- User interaction errorsIn particular the provision of “bad information” appeared as the most frequent type of failure.
- Tool errorswhere the correct tools were used incorrectly due to erroneous parameters, constituted another significant mode of failure.
A critical distinction of this reference is the categorization of errors in terminal failures (irreparable) and recoverable failures. The terminal failures are considerably more numerous than recoverable errors, illustrating the limits inherent in the auto-correction of the agent without guided intervention.
Here is an example where an agent has a “bad information”:
To meet these challenges, ATLA has integrated Selene, an evaluation model directly integrated into agent workflows. Selene actively monitors each stage of interaction, identifying and correcting errors in real time. Practical demonstrations show marked improvements when using Selene: the agents have managed to quickly correct initial errors, improving global accuracy and user experience.
Illustrated, in scenarios involving “bad information”:
- The agents operating without Selene do not regularly recover from initial errors, resulting in low satisfaction from the user.
- The agents equipped with selene have effectively identified and rectified errors, considerably improving user satisfaction and the accuracy of the responses.
Evaltoolbox thus passes manual and retrospective evaluations of errors to automated detection and immediate correction. He accomplishes this through:
- Automated categorization and identification of current failure methods.
- Real and exploitable refections when detecting errors.
- Dynamic self-correction facilitated by incorporating real-time feedback directly into the agent's work flows.
Future improvements include broader applicability through various agent functions such as coding tasks, specialized domain implementations and the establishment of standardized loop evaluation protocols.
The integration of the evaluation directly into agent work flows via the τ-bench and Evaltoolbox analysis represents a practical and automated approach to mitigate reliability problems in LLM-based agents.
Note: Thank you to the AI AI team for leadership / opinion resources for this article. The ATA AI team supported this content / article.
Asif Razzaq is the CEO of Marktechpost Media Inc .. as a visionary entrepreneur and engineer, AIF undertakes to exploit the potential of artificial intelligence for social good. His most recent company is the launch of an artificial intelligence media platform, Marktechpost, which stands out from its in-depth coverage of automatic learning and in-depth learning news which are both technically solid and easily understandable by a large audience. The platform has more than 2 million monthly views, illustrating its popularity with the public.
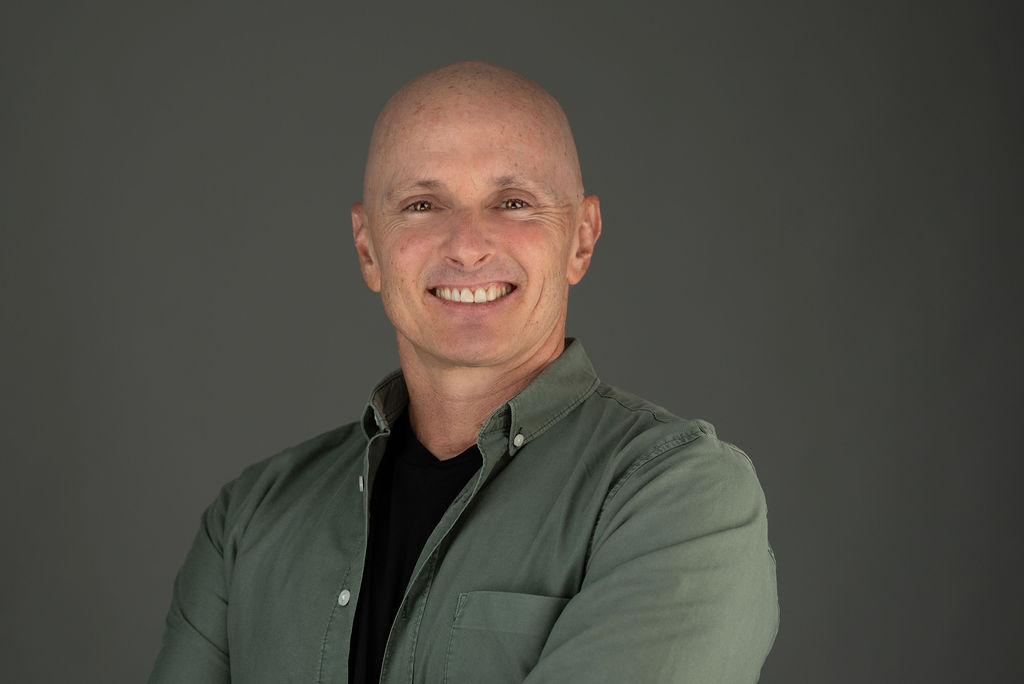