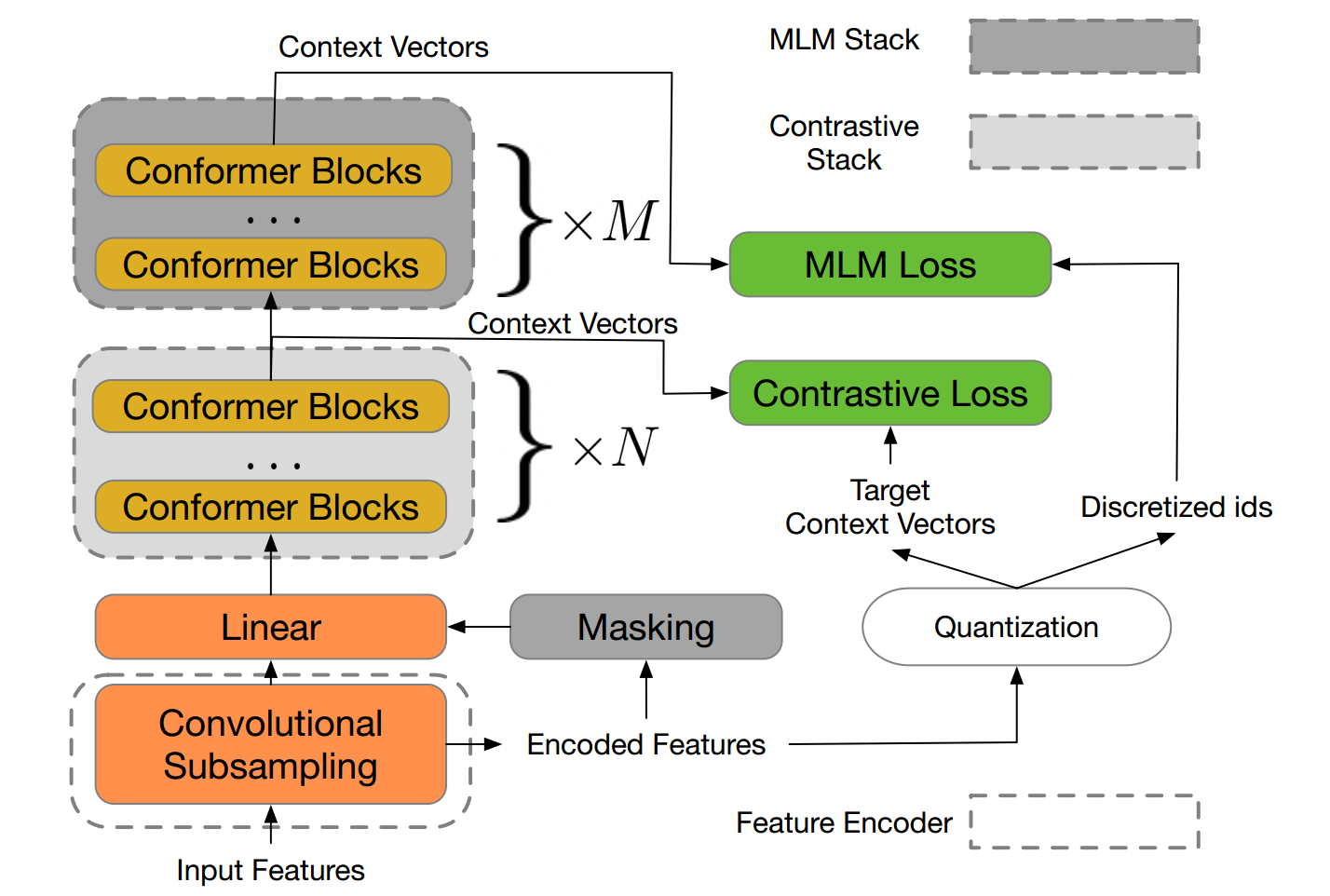
Motivated by the success of the modeling of masked languages (MLM) in the models of treatment of natural pre-training, the developers offer W2V-bert which explores the MLM for the learning of the representation of self-supervised speech.
W2V -rt is a framework that combines contrastive learning and the MLM, where the first leads to the model to discretize continuous continuous speech signals in a finite discriminating discriminative tokens, and the second leads to the model to learn from representations of contextualized speech via the resolution of a masked prediction task consuming discretized tokens.
Unlike the pre-training frameworks based on existing MLMs such as Hubert, which relies on a process of re-cluster and iterative recycling, or VQ-WAV2PEC, which concaten two modules formed separately, W2V-bert can be optimized
Experiences show that W2V-bert obtains competitive results compared to current pre-formal models on Librispeech benchmarks when using the Libri-Light ~ 60K corpus as unopensed data.
In particular, compared to published models such as WAV2W2 W1. 2.0 and Hubert based on compliance, the model represented shows a relative reduction relative of 5% to 10% on trial and test-other subsets. When applied to the data set on Google's vocal research traffic, W2V-bert surpasses our Wav2 with ~ 2.0 based on the internal compulsory of more than 30% relatively.
You can see the full article here
There is also a tutorial video On Youtube.
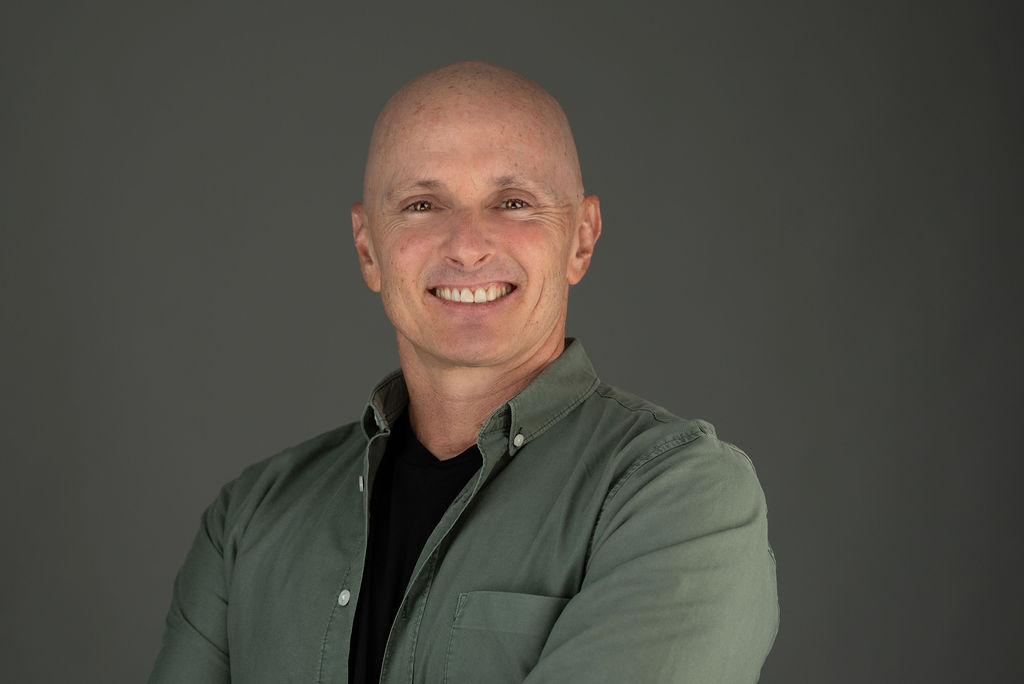