In the world of artificial intelligence, few subjects generate as many discussions and debates as the nature of large-language models (LLM) as the GPT-4 of Openai. As these models become more and more sophisticated, the question arises: are LLMs real, or are they simply gifted to simulate intelligence? To answer this, we have to immerse ourselves in what constitutes a “real” AI, the functioning of LLMs and the nuances of intelligence itself.
Define a “real” IA
Artificial intelligence (AI) is a wide term encompassing various technologies designed to perform tasks that generally require human intelligence. These tasks include learning, reasoning, problem solving, understanding natural language, perception and even creativity. AI can be classified into two main types: narrow AI and general AI.
-
Narrow: These systems are designed and trained for a specific task. Examples include recommendation algorithms, image recognition systems and, yes, LLM. The narrow AI can surpass humans in their specific fields but lack of general intelligence.
-
Ai General: This type of AI, also known as strong AI, has the ability to understand, learn and apply knowledge about a wide range of tasks, imitating human cognitive capacities. The general AI remains theoretical at this stage, because no system has reached this complete level of intelligence.
LLM mechanics
The LLM, like GPT-4, are a narrow sub-assembly. They are trained on large amounts of text data on the Internet, learning models, structures and meanings of the language. The training process consists in adjusting billions of parameters in a neural network to predict the following word in a sequence, allowing the model effectively to generate a coherent and contextually relevant text.
Here is a simplified ventilation of the operation of LLMS:
-
Data collection: LLMs are formed on various sets of data containing texts from books, articles, websites and other written sources.
-
Training: Using techniques such as supervised learning and learning to strengthen, LLM adjust their internal parameters to minimize prediction errors.
-
Inference: Once formed, LLM can generate text, translate languages, answer questions and perform other tongue -related tasks based on models learned during training.
Simulation vs authentic intelligence
The debate on the question of whether the LLMs are truly intelligent which are based on the distinction between the simulation of intelligence and possession.
-
Simulation of intelligence: LLM are incredibly able to imitate human responses. They generate text that seems reflected, contextually appropriate and sometimes creative. However, this simulation is based on the recognition of models in data rather than understanding or reasoning.
-
Intelligence: A real intelligence implies an understanding of the world, self -awareness and the ability to reason and apply knowledge in various contexts. The LLMs do not have these qualities. They do not have awareness or understanding; Their results are the result of statistical correlations learned during the training.
The Turing test and beyond
One way to assess AI is the Turing test, proposed by Alan Turing. If an AI can engage an indiscerable conversation of a human, it passes the test. Many LLM can pass simplified versions of the Turing test, which has led some to say that they are intelligent. However, criticisms underline that passing this test does not equivalent to a real understanding or conscience.
Practical Applications and Limitations
The LLMs have shown remarkable utility in various fields, from the automation of customer service to help in creative writing. They excel in tasks involving generation and understanding of languages. However, they have limits:
-
Incomprehension: LLMs do not understand the context or content. They cannot train opinions or understand abstract concepts.
-
Bias and errors: They can perpetuate the biases present in training data and sometimes generate incorrect or absurd information.
-
Data dependence: Their capacities are limited to the extent of their training data. They cannot reason beyond the models they have learned.
The LLM represents a significant progression of AI technology, demonstrating remarkable competence in the simulation of the generation of human -type text. However, they do not have real intelligence. These are sophisticated tools designed to perform specific tasks in the field of natural language treatment. The distinction between the simulation of intelligence and possession remains clear: LLM are not conscious entities capable of understanding or reasoning in the human sense. These are, however, powerful examples of narrow AI, presenting the potential and the limits of current AI technology.
While AI continues to evolve, the line between simulation and authentic intelligence can be blurred more. For the moment, the LLM testifies to the remarkable achievements possible thanks to advanced automatic learning techniques, even if they simply simulate the appearance of intelligence.
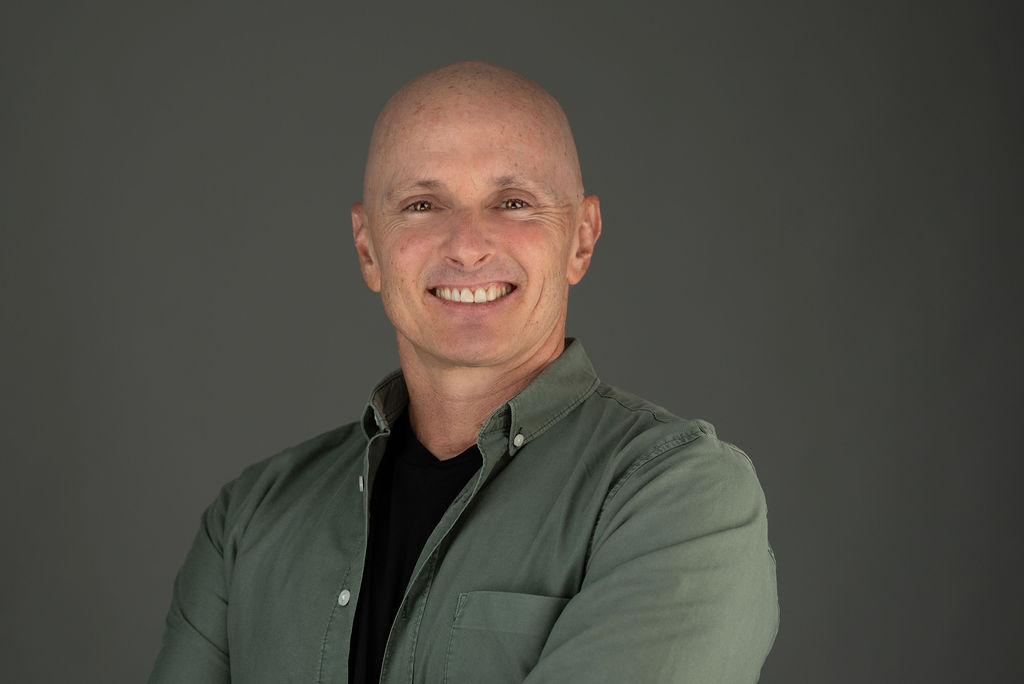