Data confidentiality has a cost. There are security techniques that protect the data from sensitive users, such as customer addresses, attackers who can try to extract them from AI models – but they often make these models less precise.
MIT researchers have recently developed a frame, based on a New confidentiality metric Called PAC Privacy, which could maintain the performance of an AI model while ensuring that sensitive data, such as medical images or financial files, remain safe from attackers. Now, they have pushed this work further by making their technique more effective on calculation, improving the compromise between precision and confidentiality, and the creation of a formal model which can be used to privatize practically any algorithm without needing access to the interior functioning of this algorithm.
The team used its new version of PAC Privacy to privatize several classic algorithms for data analysis and automatic learning tasks.
They have also shown that more “stable” algorithms are easier to privatize with their method. The predictions of a stable algorithm remain consistent even when its training data is slightly modified. Greater stability helps algorithm to make more precise predictions on previously invisible data.
The researchers say that the increased efficiency of the new PAC confidentiality framework, and the four -step model that can be followed to implement it, would make the technique easier to deploy in real situations.
“We tend to consider robustness and private life as unrelated, or perhaps even in conflict with, building a high performance algorithm. First of all, we do a work algorithm, so we make it robust, then private. We have shown that it is not always the framing. graduate student and principal author of a Document on this confidentiality framework.
She is joined in the newspaper by Hanshen Xiao Phd '24, who will start as assistant professor at Purdue University in the fall; And the main author Srini Devadas, the Edwin Professor Sibley Webster in Electric Engineering at MIT. Research will be presented at the IEEE symposium on safety and privacy.
Noise
To protect the sensitive data that has been used to form an AI model, engineers often add generic noise or random to the model so that it becomes more difficult for an opponent to guess the original training data. This noise reduces the accuracy of a model, so the less noise can add, the better.
PAC Privacy automatically estimates the smallest amount of noise you need to add to an algorithm to reach a desired level of confidentiality.
The original PAC Privacy algorithm performs a user's AI model several times on different samples of a data set. It measures the variance as well as the correlations between these many outputs and use this information to estimate the quantity of noise to add to protect the data.
This new variant of PAC confidentiality works in the same way but does not need to represent the whole matrix of data correlations between outputs; He just needs outing variances.
“Because the thing you consider is much, much smaller than the whole covariance matrix, you can do it a lot, much faster,” says Sridhar. This means that we can evolve up to much greater data sets.
The addition of noise can harm the usefulness of the results, and it is important to minimize the loss of utility. Due to the cost of calculation, the original PAC confidentiality algorithm was limited to the addition of isotropic noise, which is added uniformly in all directions. Since the new variant considers anisotropic noise, which is adapted to specific characteristics of training data, a user could add less overall noise to reach the same level of intimacy, increasing the precision of the privatized algorithm.
Confidentiality and stability
As she studied the confidentiality of the PAC, Sridhar hypothesized that more stable algorithms would be easier to privatize with this technique. It used the most effective variant of PAC confidentiality to test this theory on several classic algorithms.
More stable algorithms have less variance in their outings when their training data changes slightly. PAC Privacy divides a set of data into pieces, performs the algorithm on each piece of data and measures the variance between outputs. The larger the variance, the more noise must be added to privatize the algorithm.
The use of stability techniques to reduce the variance in the results of an algorithm would also reduce the amount of noise that must be added to privatize it, she explains.
“In the best cases, we can get these win-win scenarios,” she says.
The team has shown that these confidentiality guarantees have remained solid despite the algorithm they tested and that the new variant of PAC's confidentiality required an order of magnitude less tests to estimate noise. They also tested the method in attack simulations, demonstrating that its confidentiality guarantees could withstand advanced attacks.
“We want to explore how algorithms could be co-designed with PAC confidentiality, so the algorithm is more stable, secure and robust from the start,” explains Devadas. Researchers also want to test their method with more complex algorithms and further explore the confidentiality-unit compromise.
“The question is now: when these win-win situations occur, and how can we make them happen more often?” Said Sridhar.
“I think that the main advantage of the confidentiality of PAC has in this context on other confidentiality definitions is that it is a black box – you do not need to manually analyze each individual request to privatize the results. Wisconsin in Madison, who was not involved in this study.
This research is supported, in part, by Cisco Systems, Capital One, the American Department of Defense and a Mathworks scholarship.
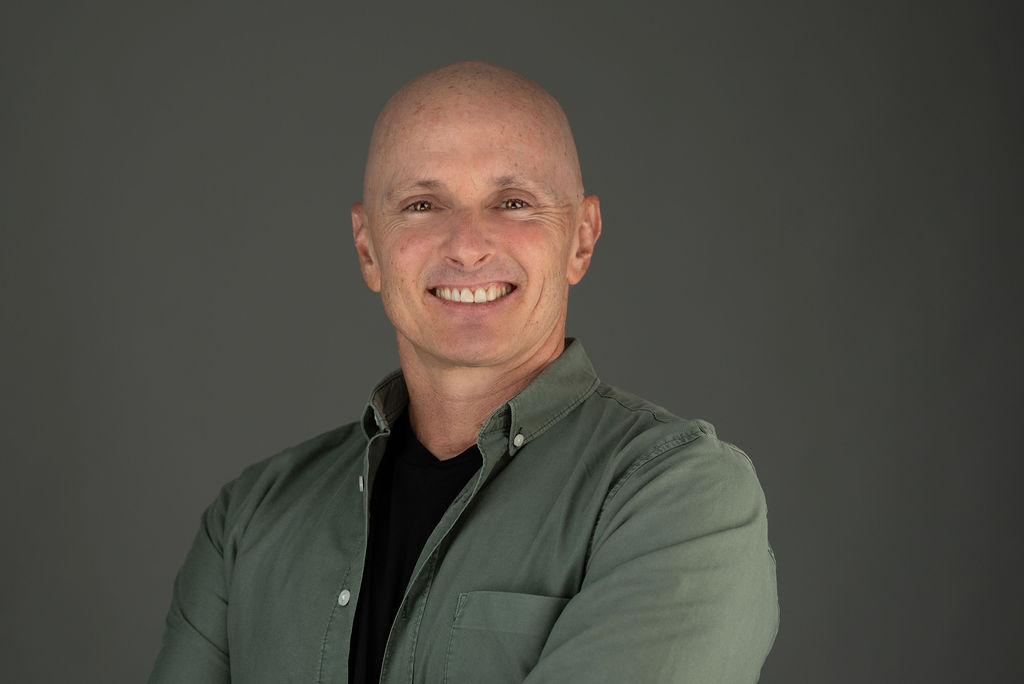