When some suburban trains arrive at the end of the line, they must go to a switching platform to be returned so that they can leave the station later, often from a different platform from that where they arrived.
Engineers use software called algorithmic solvers to plan these movements, but in a resort with thousands of arrivals and weekly departures, the problem becomes too complex for a traditional solver to disentangle.
Using automatic learning, MIT researchers have developed an improved planning system that reduces resolution time up to 50% and produces a solution that better meets the objective of a user, such as time train departures. The new method could also be used to effectively solve other complex logistical problems, such as hospital staff planning, the allocation of air teams or the allocation of tasks to factory machines.
Engineers often decompose these types of problems into a sequence of overlaps that are overlapping which can each be resolved in a possible time. But the overlaps make many decisions unnecessarily recomposed, so it takes much more time to the solver to reach an optimal solution.
The new approach improved by artificial intelligence learns which parts of each sub-problblme should remain unchanged, freezing these variables to avoid redundant calculations. Then, a traditional algorithmic solver addresses the remaining variables.
“Often, a dedicated team could spend months or even years, to design an algorithm to solve only one of these combinatorial problems. Deep learning modern gives us the opportunity to use new advances to help rationalize the design of these algorithms. We can take what we know well, and use AI to accelerate computer science, “said Cathy Wu, The Capyal, Cabot Associated Cariel) and Cabot) and Caullage) and the Cabot Development Cariel and Caaugeing) and the Cauria W. Institute for data, systems and company (IDSS) (IDSS). (Lids).
She is joined on the paper by the main author Sirui Li, an IDSS graduate student; Wenbin Oulang, a student graduated from the EEC; And Yining MA, a postdoc lid. Research will be presented at the international conference on representations of learning.
Eliminate redundancy
A motivation for this research is a practical problem identified by a Devin Camille Wilkins master's student in the entry -level transport course in WU. The student wanted to apply for a real train dopatch problem at the northern Boston station. The public transport organization must assign many trains to a limited number of platforms where they can be returned long before their arrival at the station.
This turns out to be a very complex combinatorial planning problem – the exact type of problem that the WU laboratory has spent in recent years working.
Faced with a long -term problem which involves awarding a limited set of resources, such as factory tasks, a group of machines, planners often solve the problem as a flexible job workshop planning.
In the flexible planning of the job workshop, each task needs a different delay, but the tasks can be assigned to any machine. At the same time, each task is made up of operations which must be carried out in the right order.
Such problems quickly become too large and too heavy for traditional resolors, so that users can use the optimization of Rolling Horizon (RHO) to divide the problem into manageable pieces which can be solved more quickly.
With RHO, a user attributes a few first tasks to machines in a fixed planning horizon, perhaps a four-hour window. Then, they perform the first task of this sequence and move the four -hour planning horizon forward to add the following task, repeating the process until the whole problem is solved and the final calendar of task machine assignments is created.
A planning horizon must be longer than the duration of a task, because the solution will be better if the algorithm also considers the tasks that will happen.
But when the planning horizon progresses, this creates some overlap of operations in the previous planning horizon. The algorithm has already offered preliminary solutions to these overlapping operations.
“Perhaps these preliminary solutions are good and don't need to be calculated, but perhaps they are not good. This is where automatic learning comes into play, ”explains Wu.
For their technique, which they call the optimization of the rolling horizon guided by learning (L-RHO), the researchers teach an automatic learning model to predict which operations, or variables, should be recompuous when the planning horizon rolls forward.
The L-RHO requires data to form the model, so the researchers solve a set of sub-problublicms using a classic algorithmic solver. They have taken the best solutions – those that have the most operations that do not need to be recomposed – and used them as training data.
Once formed, the automatic learning model receives a new sub-problblme that he has never seen before and predicts which operations should not be recomposed. The remaining operations are returned to the algorithmic solver, which performs the task, rewards these operations and advances the planning horizon. Then the loop starts again.
“If, with hindsight, we did not need to restore them, then we can remove these variables from the problem. Because these problems increase exponentially in size, this can be quite advantageous if we can drop some of these variables,” she adds.
An adaptable and scalable approach
To test their approach, the researchers compared the L-RHO to several basic algorithmic solvers, specialized solvents and approaches that only use automatic learning. This has outperformed them, reducing the resolution time by 54% and improving the quality of the solution up to 21%.
In addition, their method continued to surpass all the basic lines when they tested it on more complex variants of the problem, such as when factory machines decompose or in the event of additional train congestion. He even surpassed the additional basic lines that researchers have created to challenge their solver.
“Our approach can be applied without modification to all these different variants, which is really what we started to do with this search line,” she says.
L -RHO can also adapt if the objectives change, automatically generating a new algorithm to solve the problem – everything it needs is a new set of training data.
In the future, researchers want to better understand the logic behind the decision of their model to freeze certain variables, but not others. They also want to integrate their approach into other types of complex optimization problems such as stock management or vehicle routing.
This work was supported, in part, by the National Science Foundation, the MIT research support committee, a dozon and Mathworks doctoral scholarship.
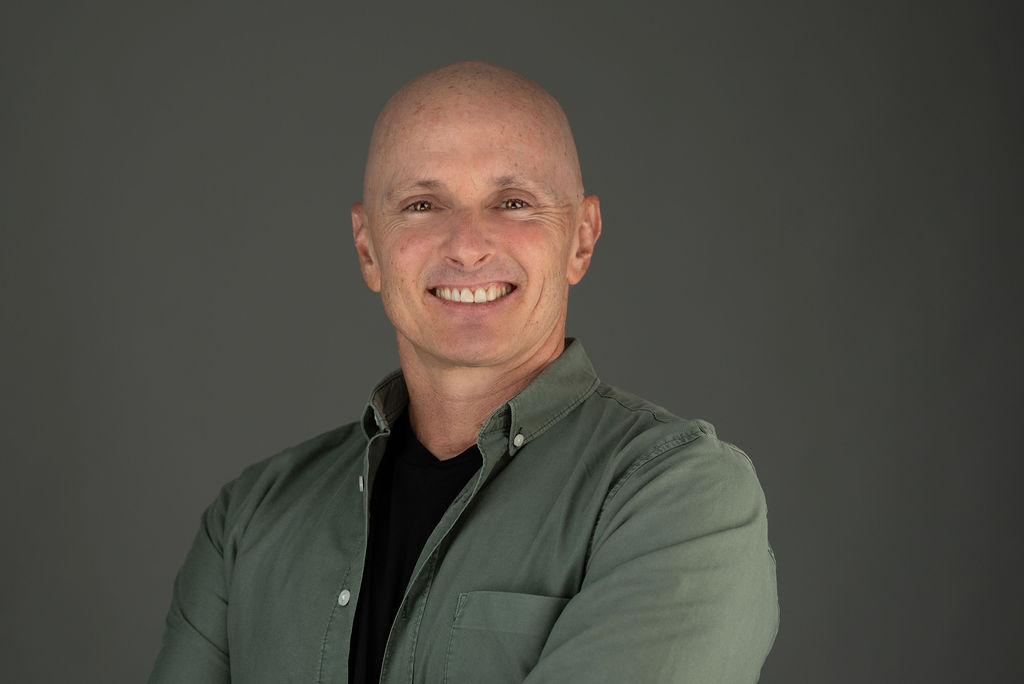