The use of the modern web covers many digital interactions, the filling of forms and the management of the accounts to the execution of data requests and the navigation on complex dashboards. Although the web is deeply linked to productivity and work processes, many of these actions still require a repetitive human contribution. This scenario is particularly true for environments that require detailed instructions or decisions beyond simple research. While the agents of artificial intelligence have emerged to support the automation of tasks, many hierarchize complete autonomy. However, this frequently sets out to use user control, leading to results that diverge user expectations. The next leap forward in the IA improving productivity involves agents designed so as not to replace users but to collaborate with them, mixing automation with a continuous and real -time human entry for more precise and reliable results.
A key challenge in the deployment of AI agents for tasks on the web is the lack of visibility and intervention. Users often cannot see what steps the agent is planning, how it intends to execute them or when it can disconnect. In scenarios that involve complex decisions, such as seizure of payment information, interpretation of dynamic content or script execution, users need mechanisms to intervene and redirect the process. Without these capacities, systems may make irreversible mistakes or to disalmark with user objectives. This highlights a significant limitation of the automation of current AI: the absence of human structured conception in a loop, where users dynamically guide and supervise the behavior of agents, without acting simply as spectators.
Previous solutions have discussed web automation via scripts based on rules or AI agents for general use piloted by language models. These systems interpret user commands and try to make them independently. However, they often perform plans without surfaceing intermediate decisions or allow significant comments. Some offer command form interactions, which are inaccessible to the average user and rarely include layering safety mechanisms. In addition, a minimum support for the reuse of tasks or learning performance between sessions limits the long -term value. These systems also tend to lack adaptability when the context changes in the middle of the task or that errors must be corrected in collaboration.
Microsoft researchers presented Magentic-iAn open source prototype that emphasizes human-AI collaborative interaction for web tasks. Unlike previous systems aimed at complete independence, this tool promotes real-time co-layout, execution and surveillance of user step by step. Magentic-Iu is built on the Autogen frame of Microsoft and is closely integrated into Azure Ai Foundry Labs. It is a direct evolution of the previously introduced Magentic-One system. With its launch, Microsoft Research aims to answer fundamental questions about human surveillance, safety mechanisms and learning in agency systems by offering an experimental platform for researchers and developers.
Magentic-IU has four basic interactive characteristics: co-plague, co-tashes, action guards and learning the plan. Co-platation allows users to display and adjust the stages proposed by the agent before the start of the execution, offering total control over what the AI will do. Co-tasse allows real-time visibility during operation, allowing users a break, modify or take charge of specific actions. The action guards are customizable confirmations for high -risk activities such as closing the browser tabs or click on “Submit” on a form, actions that could have unexpected consequences. Learning the plan allows Magentic-Ui to remember and refine the steps for future tasks, improving time thanks to experience. These capacities are supported by a modular team of agents: the orchestrator leads planning and decision -making, websurfer browser interactions, the coder performs code in a sandbox and filesurner interprets files and data.
Technically, when a user submits a request, the orchestrator agent generates a step -by -step plan. Users can modify it via a graphical interface by modifying, deleting or regeneration of the steps. Once finalized, the plan is delegated to specialized agents. Each agent reports after having carried out his task and the orchestrator determines whether to proceed, repeat or request user comments. All actions are visible on the interface and users can stop execution at any time. This architecture guarantees not only transparency, but also allows adaptive task flows. For example, if a step fails due to a broken link, the orchestrator can dynamically adjust the plan with the consent of the user.
In controlled evaluations using the GAIA reference, which includes complex tasks such as web browsing and interpretation documents, Magentic-Iu's performance have been rigorously tested. Gaia consists of 162 tasks requiring a multimodal understanding. During the functioning independently, Magentic-Iu succeeded 30.3% of the tasks successfully. However, when supported by a simulated user with access to additional task information, success increased to 51.9%, an improvement of 71%. Another configuration using a smarter simulated user improved the rate at 42.6%. Interestingly, Magentic-Iu asked for help only in 10% of improved tasks and asked for final responses in 18%. In these cases, the system has requested an average aid of only 1.1 times. This shows how timed minimal but well timed human intervention considerably increases the completion of tasks without high surveillance costs.
Magentic-Iu also offers a “recorded plans” gallery which displays reused strategies of past tasks. The recovery of this gallery is about three times faster than the generation of a new plan. A predictive mechanism surfaces these plans while users hit, rationalizing repeated tasks such as flight research or form submissions. The safety mechanisms are robust. Each browser or code action runs in a Docker container, ensuring that no user information is exposed. Users can define authorization lists for access to the site, and each action can be closed behind approval prompts. An assessment of the Red team has also tested it against phishing attacks and rapid injections, where the system requested user clarification or blocked the execution, strengthening its diaper defense model.
Several key dishes of research on Magentic-ii:
- With a simple human entry, Magentic-Iu increases the completion of the 71% task (from 30.3% to 51.9%).
- Requests of user help in only 10% of improved tasks and on average 1.1 requests for assistance per task.
- It has a co-plain user interface which allows complete control of the user before execution.
- Performs tasks via four modular agents: orchestrator, Websurfer, Coder and Filesurfer.
- Stores and reuse plans, reducing repeated task latency up to 3x.
- All actions are in sandbox via Docker containers; No user information is never exposed.
- Adopted red team assessments against phishing and injection threats.
- Supports the “action guards” entirely configurable by the user for high -risk steps.
- Completely open-source and integrated into the Azure Ai Foundry laboratories.
In conclusion, Magentic-Iu addresses a long-standing problem in AI automation, the lack of transparency and controllable. Rather than replacing users, it allows them to stay at the heart of the process. The system works well even with a minimum help and learns to improve each time. Modular design, robust guarantees and detailed interaction model create a solid base for future smart assistants.
Discover the Technical details And GitHub page. All the merit of this research goes to researchers in this project. Also, don't hesitate to follow us Twitter And don't forget to join our 95K + ML Subdreddit and subscribe to Our newsletter.
Asif Razzaq is the CEO of Marktechpost Media Inc .. as a visionary entrepreneur and engineer, AIF undertakes to exploit the potential of artificial intelligence for social good. His most recent company is the launch of an artificial intelligence media platform, Marktechpost, which stands out from its in-depth coverage of automatic learning and in-depth learning news which are both technically solid and easily understandable by a large audience. The platform has more than 2 million monthly views, illustrating its popularity with the public.
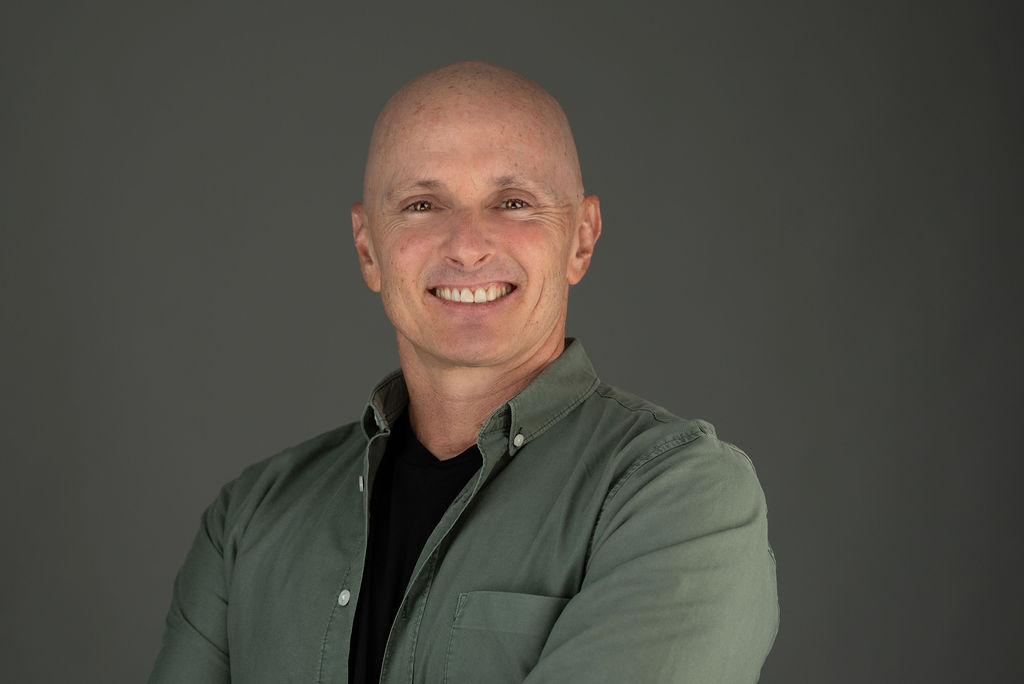