Large models of reasoning (LRM) such as O1 and O3 of Openai, Deepseek-R1, Grok 3.5 and Gemini 2.5 Pro have shown strong capacities in long-COT reasoning, often displaying advanced behaviors such as self-correction, backwards and verification-known in the collection under the name of “AHA moments”. It was observed that these behaviors emerge thanks to the RL results on the results without the need for a supervised fine adjustment. Models like Deepseek -R1 and its open source replications (for example, Tinyzero and Logic -RL) have shown that carefully designed RL pipelines – using rules based on rules, learning curriculum and structured training – can induce such reflective reasoning capacities. However, these emerging behaviors tend to be unpredictable and inconsistent, which limits their practical reliability and scalability.
To remedy this, the researchers explored structured RL frames which target specific types of reasoning, such as deduction, abduction and induction. These approaches involve align specialized models, merge them into the parameters space and apply a continuous RL specific to the domain. Tools such as logic-RL use an RL conditioned RL rules to resolve logical puzzles, improving transferability to tasks such as mathematical reasoning. Meanwhile, other works offer mechanisms to improve the robustness of reasoning, such as training models to reason both in front and back, or to self -line their results. Studies analyzing the “AHA moments” suggest that these behaviors come from internal changes in uncertainty, latent representation and self-assessment, offering new information on engineering of more reliable reasoning models.
Researchers from the National University of Singapore, the University of Tsinghua and the research on the AI of Salesforce deal with limits to rely on spontaneous “AHA” in large language models by explicitly aligning them with three basic reasoning capacities: deduction, induction and abduction. They introduce a three -step pipeline – alignment of individual meta -capacity, fusion of parameter space and specific reinforcement learning – considerably improving model performance. Using a series of self-verifiable tasks generated by program, their approach increases accuracy compared to the reference lines set by the instruction by more than 10%, with other RL gains specific to the domain. This structured alignment framework offers an evolutionary and generalizable method to improve reasoning through mathematical, coding and scientific fields.
The researchers have designed tasks aligned with the deduction, induction and abduction using a structured format “given two, deduce the third” based on the hypothesis (H), the rule (R) and the observation (o). The deduction is considered to be a verification of satisfied, induction as a prediction of masked sequence and abduction as the opposite inference of the rules of the rules. These tasks are generated synthetically and automatically verified. The training pipeline includes three stages: (a) independent training models for each type of reasoning using RealForce ++ with structured rewards, (b) the fusion of models by interpolation of weighted parameters and (C) the fine adjustment of the unified model on the data specific to the field via reinforcement learning, the isolation of the alignment of the alignment of meta-capacity.
The study assesses the models aligned with meta -capacity – detection, induction and abduction – using a program learning configuration through difficulty levels. The models formed on synthetic tasks are strongly generalized with seven benchmarks of mathematics, codes and invisible sciences. On scales 7b and 32b, the models aligned and merged on meta-capacity constantly surpass the base lines in instruction, the merged model offering the highest gains. The RL specific to the continuous domain of these merged control points (Domaine-RL-META) leads to additional improvements compared to the standard RL FINDUNING (Domaine-RL-IN), in particular in mathematical references. Overall, the alignment strategy improves reasoning capacities, and its scale of advantages with the size of the model, considerably increasing the performance ceilings between tasks.

In conclusion, the study shows that large models of reasoning can develop advanced problem solving skills without depending on unpredictable “AHA moments”. By aligning the models with three basic reasoning capacities – the eduction, induction and abduction, using auto -verifiable tasks, the authors create specialized agents which can be effectively combined in a single model. This merged model surpasses the reference bases set by the instruction of more than 10% on diagnostic tasks and up to 2% on real references. When used as a starting point for learning specific strengthening in the field, it increases performance by 4%. This modular and systematic training approach offers an evolutionary and controllable basis to build reliable and interpretable reasoning systems.
Discover the Paper And GitHub page. All the merit of this research goes to researchers in this project. Also, don't hesitate to follow us Twitter And don't forget to join our 95K + ML Subdreddit and subscribe to Our newsletter.
Sana Hassan, consulting trainee at Marktechpost and double -degree student at Iit Madras, is passionate about the application of technology and AI to meet the challenges of the real world. With a great interest in solving practical problems, it brings a new perspective to the intersection of AI and real life solutions.
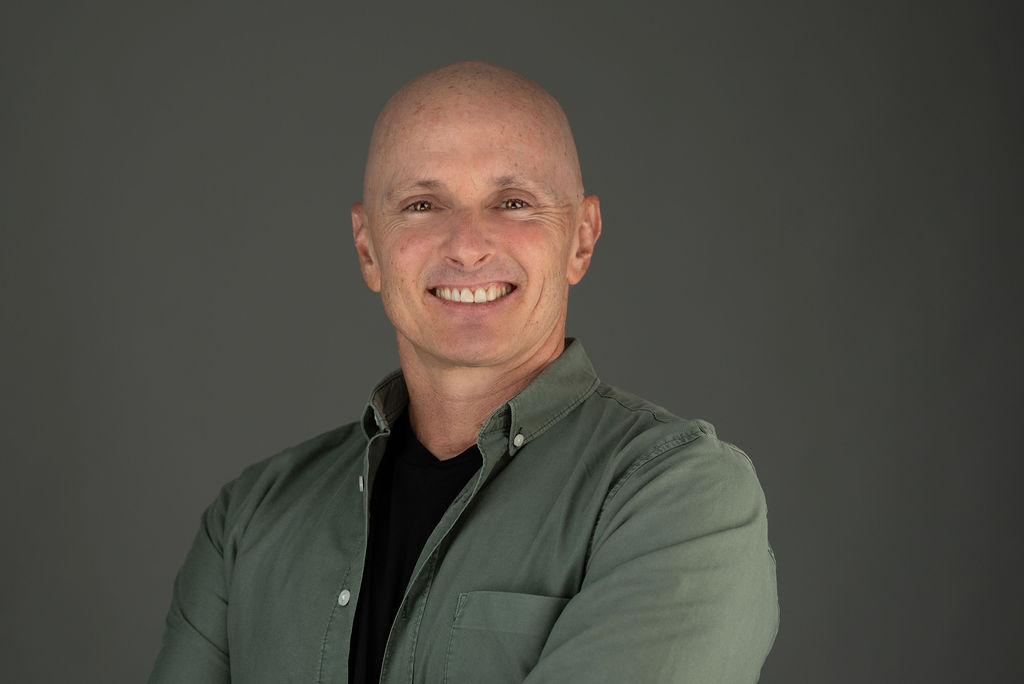