Language models (LMS) have large capacities as a context in context when they are pre-trained on large corpus of internet text, allowing them to generalize effectively from a few examples of tasks. However, the fine adjustment of these models for downstream tasks has important challenges. Although the fine setting requires hundreds to thousands of examples, the resulting generalization models show limitations. For example, the models have refined declarations like “B East” the mother “to answer related questions like” who is the son of an A? ” However, the LMS can manage such inverted relationships in the context. This raises questions about the differences between learning in the context and fine generalization models, and how these differences should shed light on adaptation strategies for downstream tasks.
Research on improving LMS adaptability has followed several key approaches. Learning studies in context have examined learning and generalization models through empirical, mechanistic and theoretical analyzes. Executive learning research explores how models use information that is not explicitly included in the prompts. Data increased techniques use LLM to improve the performance of limited data sets, with specific solutions targeting problems such as inversion curse by hard coded increases, deductive closing formation and the generation of reasoning routes. In addition, synthetic data approaches have gone from data designed by the first hands to improve generalization in fields such as linguistics or mathematics to more recent methods that generate data directly from linguistic models.
Researchers from Google Deepmind and the University of Stanford have built several data sets that isolate knowledge from pre-training data to create clean generalization tests. Performance is evaluated on various types of generalization by exposing pre-extended models to subsets of controlled information, both in the context and by a fine adjustment. Their results reveal that learning in the context shows a more flexible generalization than the fine adjustment in the matching parameters of data, although there are some exceptions where the fine adjustment can be generalized in reversions in more important knowledge structures. Based on these ideas, the researchers have developed a method that improves the generalization of the fine adjustment by including the inferences in context in the fine adjustment data.
Researchers use several data sets carefully designed to isolate specific generalization challenges or insert them into wider learning contexts. The evaluation is based on the rating of the multiple probability of choice without providing any choice of response in context. Experiences involve a gemini 1.5 flash with fine adjustment using 8 or 16 lots of lots. For the assessment in context, researchers combine training documents as well as context for the investigation model, underlying random by 8x for larger data sets to minimize interference problems. Key innovation is an approach to increase the data set using generalization in the context to improve coverage of the fine adjustment data set. This includes local and global strategies, each using separate contexts and prompts.
On the confermers of the inversion curse, learning in context achieves almost ceiling performance on inversions, while the conventional end adjustment shows a precision close to zero, because the models promote incorrect celebrities names observed during the training. The fine adjustment with data increased by contexts in context corresponds to the high performance of pure learning in the context. Tests on simple non-sensations reveal similar models, but with less pronounced advantages. For simple syllogisms, although the pre-trained model works at the level of chance (indicating no contamination of data), a fine adjustment produces a generalization above luck for certain types of syllogism where logical inferences align with simple linguistic models. However, learning in the context surpasses the fine adjustment, with an increased fine adjustment showing the best overall results.
In conclusion, this article explores the differences in generalization between learning in context and fine adjustment when the LMS faces new information structures. The results show the higher generalization of learning in context for certain types of inference, which has prompted researchers to develop methods that improve fine adjustment performance by incorporating contextual inferences into training data. Despite promising results, several limitations affect the study. The first is the dependence of absurd words and incredible operations. Second, research focuses on specific LMs, limiting the generality of the results. Future research should study the differences in learning and generalization between different models to develop these results, in particular new models of reasoning.
Discover the Paper. All the merit of this research goes to researchers in this project. Also, don't hesitate to follow us Twitter And don't forget to join our 95K + ML Subdreddit and subscribe to Our newsletter.
Sajjad Ansari is a last year's first year of the Kharagpur Iit. As a technology enthusiast, he plunges into AI's practical applications by emphasizing the understanding of the impact of AI technologies and their real implications. It aims to articulate complex AI concepts in a clear and accessible way.
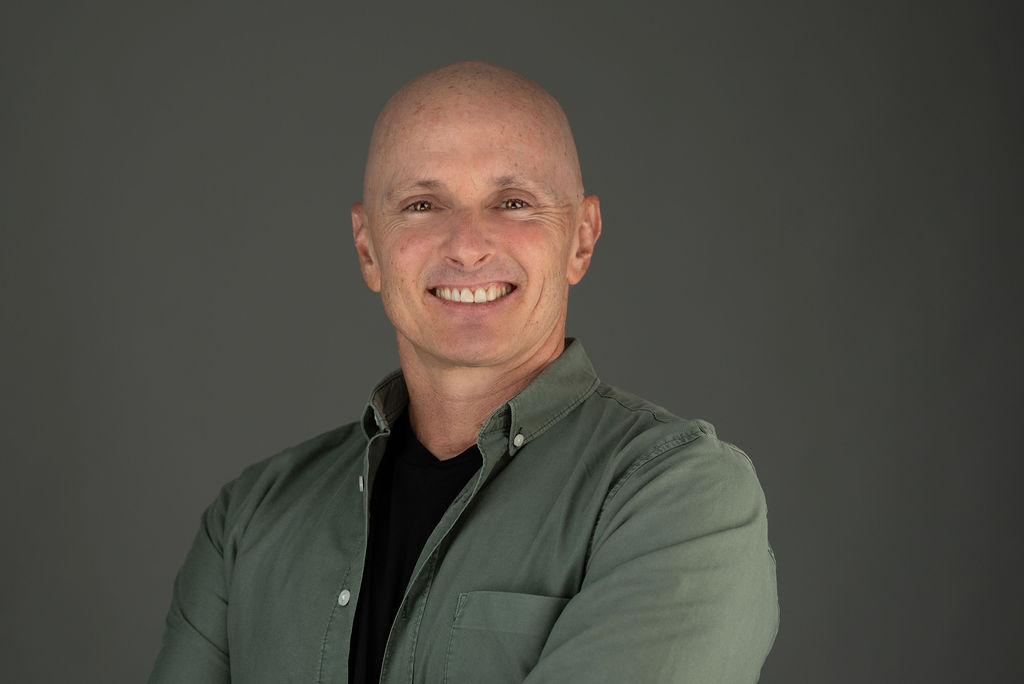