Great languages (LLM) models have drawn significant attention in recent years, but understanding their internal mechanisms remains difficult. When examining individual attention heads in models of transformers, the researchers identified specific features in certain heads, such as induction heads which predict tokens as “Potter” following “Harry” when the sentence appears in its context. Ablation studies confirm the causal relationship of these chiefs to model behavior. However, most heads of attention distribute the development in various contexts without clear functionality. The challenge lies in the interpretation of these complex attention models, because inter-heady collaboration often occurs rather than isolated features. This phenomenon resembles a superposition in neuronal interpretation, suggesting the existence of a superposition of attention in the mechanisms of multiple self-attuction self-attuuation (MHSA). Understanding these complex interactions is crucial to develop more transparent and controllable language models.
Previous research has made significant progress to explain the individual head of attention by using techniques such as activation fix and path fix. These approaches have identified several attention heads specializing in models of transformers, including composition heads, induction heads, name moving heads, number comparison heads, copy deletion heads, successor heads and long context recovery heads. However, the superposition hypothesis suggests that neurons are linked to several non-orthogonal underlying characteristics rather than unique features. Large self-employed people have become a promising method for extracting over-complets sets from sparse and linearly understandable features from neural networks. The success of these self -entertainment demonstrates the universality of superposition through different dimensions, including the size of the model, the types of architecture and even the different methods. These methods, although precious, still find it difficult to fully explain the complex interactions between the heads of attention and their collaborative behavior in language models.
The search for Shanghai Innovation Institute, OpenMoss Team, School of Computer Science, Fudan University Low light sparse attention (when)A robust approach to unravel the atomic attention units of the superposition of attention. At the time of replacing the self-tension with several standard heads with a set of attention heads on complete which include single OV circuits and rarity constraints. To assess at the same time, the researchers have developed an exploration interface which provides complete information on each head of ETAA, quantitatively assessing interpretability through activation and allocation models. The results demonstrate that the monosecticity of ESA is compared favorably to the characteristics of the sparse self -dencoder. The method was tested on the Pythia-160m and Llama-3.1-8B models, successfully identifying known attention mechanisms such as induction heads, moor heads, successful heads and attention wells. A more in-depth analysis has revealed specific heads specific to arithmetic in LLAMA-3.1-8B and identified thematic anchor heads with long-range and specific attention models on the subject. This approach offers unprecedented visibility in the attention mechanisms of transformers.
The superposition of attention in the models of transformers is parallel to the way in which neurons represent more characteristics than their dimensions. Research issues the hypothesis that MHSA includes multiple attention units in superposition, each participating in specific token pairs with interpretable reading / writing operations on the residual flow. This hypothesis suggests that atomic attention units propagate on several MHSA heads, while individual heads contain several units.
Three key evidence support the superposition of attention: firstly, polytentic heads respond to unrelated entries, such as successive heads that increment on days, numbers and acronyed behaviors / copy simultaneously. Secondly, most of the attention heads lack clear interpretation patterns, studies showing attempts to interpret failed for more than 90% of GPT-2 heads. Third, direct observations show that the attention exit characteristics have collectively contributed by several heads, with around 25% of learned attention units spread over several MHSA heads.
Clearly understand the superposition of attention for two key reasons. First, the circuit tracing based on the allocation becomes difficult when the functionalities are calculated collectively, because the models of individual requests can be induced due to the interference of other features in the same heads. Second, the structure of the superposition of attention can reveal important reasons for models of models, which raises questions about the reasons why certain units of attention, such as induction heads, are implemented by unique MHSA heads while others exist in superposition.
The architecture at the timea takes up these challenges through several innovative design elements. At the time of predicting MHSA outputs by minimizing the average quadratic error. It uses one -dimensional OV circuits that restrict reading / writing operations to specific residual flow characteristics, aligning with the hypothesis of linear representation. For requests and key weights, when implements the sharing of parameters on each Dlorsa QK head, maintaining the effectiveness of the parameters while preserving performance. This strategy makes circuits at QK similar to MHSA but with constraints of rarity on each OV dimension.
When it uses more heads than the standard MHSA while activating only a small subset per token. For each position, the output of ETHAs only fell the upper heads with the most important activation values, the active head subset varying dynamically through the token positions. This approach looks like Topk-Sae, by selecting the most salient linear components. Although similar to freight autoencoders at attention, the at the time of differs in that its head activations derive from the attention models of previous tokens rather than simple linear encoders with relu.
The evaluation of the interpretability of ESA uses several key measures to understand the individual head features. The main activations help identify the models by examining the 16 highest activation tokens for each head of at 100 million samples from held data. The analysis of the Z pattern decomposes the activations linearly into contributions to token from previous positions, revealing which previous tokens contribute to current activations. This approach is parallel to the allocation analysis of the direct characteristics used for the attention of sparse self -entertainment, but with a simpler attribution involving a single one -dimensional OV circuit and a single QK circuit.
A visualization dashboard provides complete information on each head of at of course. For example, an induction head specific to “you” shows several important models: it mainly reads features indicating that the current token is “you” / “Your” through its weight vector, strongly active a “Say You” functionality which amplifies the logit of “you” and increases the probabilities of prediction for various “you” tokens. The calculation of the QK attention model implies current token features in the request for a query and preceding token functionalities where the current token is “you”, the previous token is often words like “with”, “thank you” or “do”. Interestingly, this particular head of Enda is almost also distributed between two MHSA heads (5.0 and 5.7), demonstrating how when the units of attention that exist in several standard attention heads successfully.
The results confirm the efficiency of ETA in the identification of the attention mechanisms known on different models. Using the path patch, the researchers rediscovered monosemantic heads previously documented at Pythie-160m, including induction heads, names of names, copy deletion heads, successor heads and attention wells. In LLAMA-3.1-8B, they identified ARISA heads specific to arithmetic which are activated during simple arithmetic operations, each head using separate heuristics to recover operands. In addition to that, they discovered thematic anchor heads which have long -term attention to the topically linked tokens, suggesting a mechanism to maintain representations of persistent subjects which bias the predictions of subsequent token towards vocabulary and structures appropriate by the field.
Low light sparse attention Successfully designs the atomic attention units of the superposition of attention in the models of transformers. The method effectively recovers known attention mechanisms while discovering new interpretable behaviors, demonstrating its value for the interpretability of the neural network. Despite these advances, significant challenges remain in the QK circuits not linked to the realization of the entirely independent heads and the reduction of the superposition effects. Future research guidelines include exploration of low -dimension QK structures, transverse superposition and systematic Q / K / V composition.
Discover the Paper,, Model on the embraced face And GitHub page. Also, don't forget to follow us Twitter.
Here is a brief overview of what we build on Marktechpost:
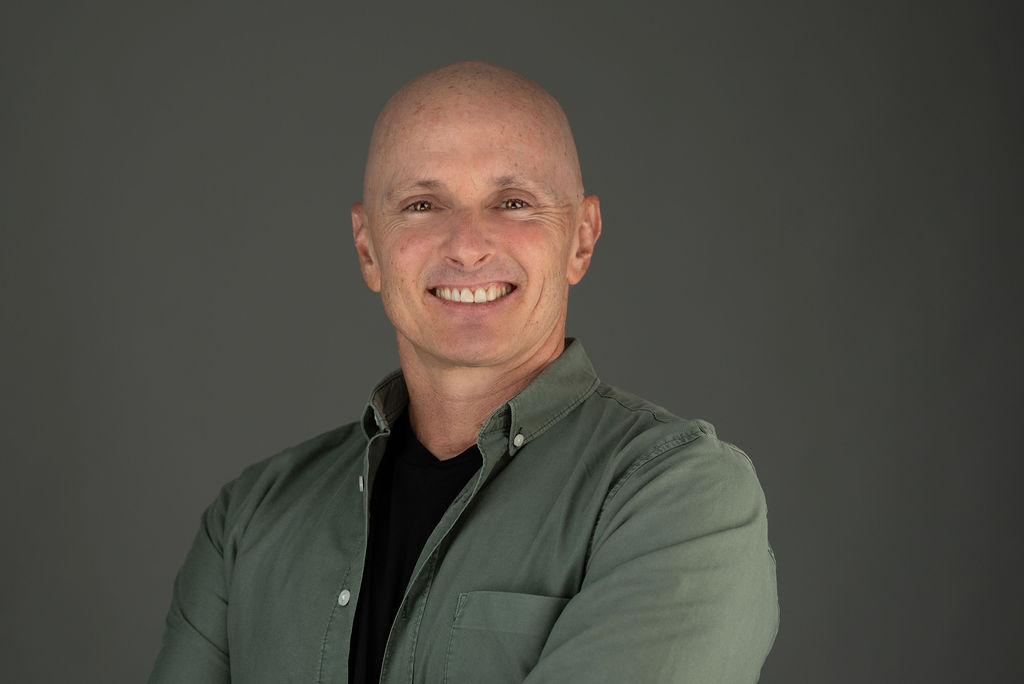