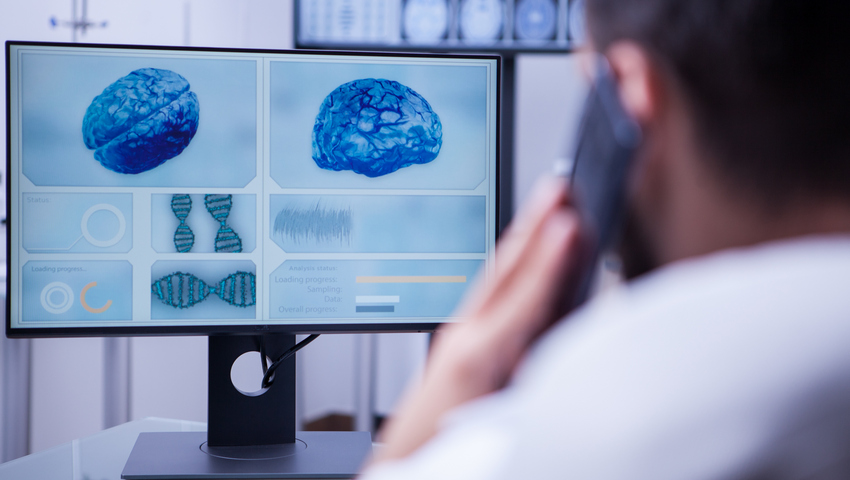
MIT and Dana-Farber Cancer Institute researchers have designed A new method to take up the challenge of identifying cancer origin in a subset of patients. This challenge occurs when doctors are unable to determine the source of cancer, which makes it difficult to determinate the most appropriate treatment, as many cancer drugs are suitable for specific types of cancer.
The newly developed approach uses automatic learning and implies the creation of a calculation model. This model can analyze the genetic sequence of around 400 genes and use this information to predict the source of a particular tumor in the body.
Thanks to this method, the team has managed to classify more than 40% of tumors with an unknown origin in an unknown origin containing around 900 patients. This breakthrough has enabled a remarkable increase of 2.2 times to the number of patients who could potentially be candidates for personalized and genomic guided treatments, depending on the identified source of their cancer.
Intae Moon, the main study of the study and student graduated in electrical engineering and IT engineering, stressed the significant conclusion that the model could potentially help health professionals to make treatment decisions by guiding them to personalized therapies for patients with unknown primary cancer.
Alexander Gusev, the main author of the newspaper and an associate professor of medicine at the Harvard Medical School and the Dana-Farber Cancer Institute, underlined the impact of this work, especially on people with unknown primary cancers, a disease affecting approximately 3 to 5% of patients with cancer.
Traditionally, the lack of knowledge concerning the main original cancer site prevented doctors from administering targeted treatments. These treatments, adapted to specific types of cancer, are often more effective and have fewer side effects than the generalized treatments prescribed for a wide range of cancers.
The study methodology focused on analyzing the genetic data collected regularly from Dana-Farber. The data included genetic sequences of around 400 genes commonly transferred to cancer. Researchers have formed an automatic learning model using data of nearly 30,000 patients with 22 types of known cancer. Subsequently, this model, named onconpc, was tested on approximately 7,000 tumors previously invisible with known origins. It has demonstrated an accuracy rate of around 80%, which reached approximately 95% for high trust forecasts.
On these promising results, the model was applied to a set of data of around 900 tumors of individuals with cancers of unknown primary origin. The model has successfully generated high trust forecasts for 40% of these cases.
The predictions of the model were validated by comparing them with the analysis of germ mutations in a subset of tumors. The predictions of the model are often aligned with the type of cancer predicted by these genetic mutations. In addition, the predictions of the model have been aligned with patient survival times and their responses to treatments.
By allowing the identification of the source of cancer, the researchers actually expanded the pool of patients who could benefit from targeted treatments which were already available. Research was supported by various foundations, including the National Institutes of Health and the Louis B. Mayer Foundation.
In the future, researchers aim to improve their model by incorporating additional data types, such as pathology and radiology images, to provide a more complete prediction encompassing various data methods. This could allow the model not only to predict the types of tumors and the results for patients, but also recommends optimal treatment strategies.
Automatic learning techniques have often been used by scientists to detect diseases, presenting their versatility and their potential in the field. The Qudata team has further informed the essential role of AI and automatic learning in health care thanks to various projects. Our team has made significant progress in the progress of medical diagnostics, especially in the field of Cervical column fracture detection. Our innovative solution in complex combines advanced image processing methodologies and automatic learning models to discern fractures of the cervical column of CT scans. This pioneering approach, based on the analysis of the bone structure and density, allows a precise location of fracture sites. Consequently, health professionals can quickly and accurately diagnose fractures, predetermination of potential complications and optimization of patient care.
For an in -depth exploration of the efforts and achievements of Qudata, read Qudata AI / ML case studieswhich highlighted the revolutionary work carried out by our team.
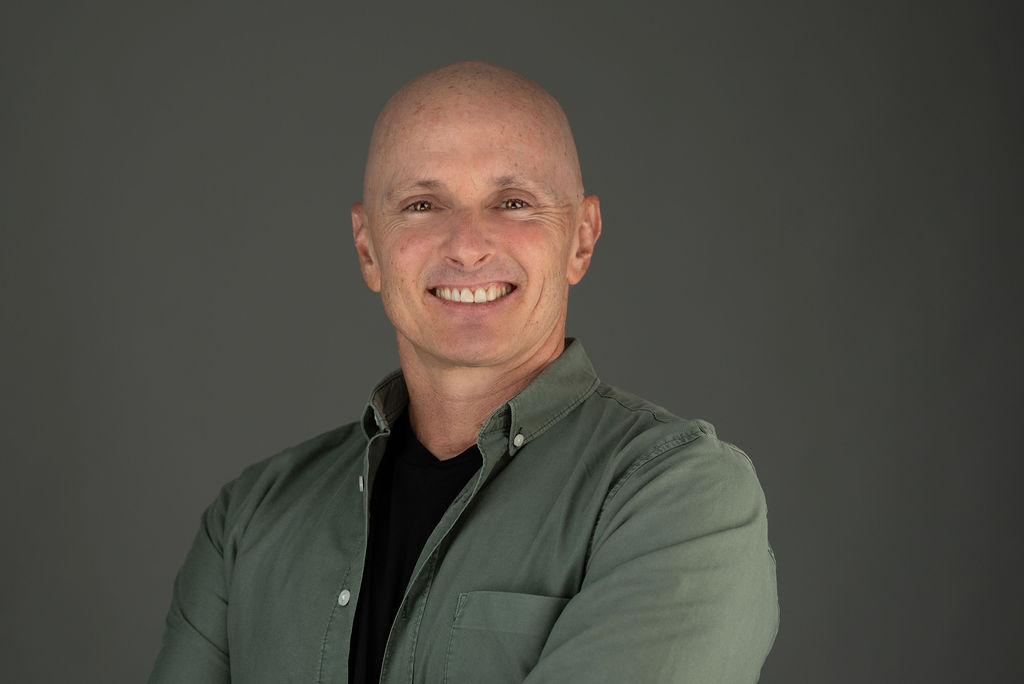