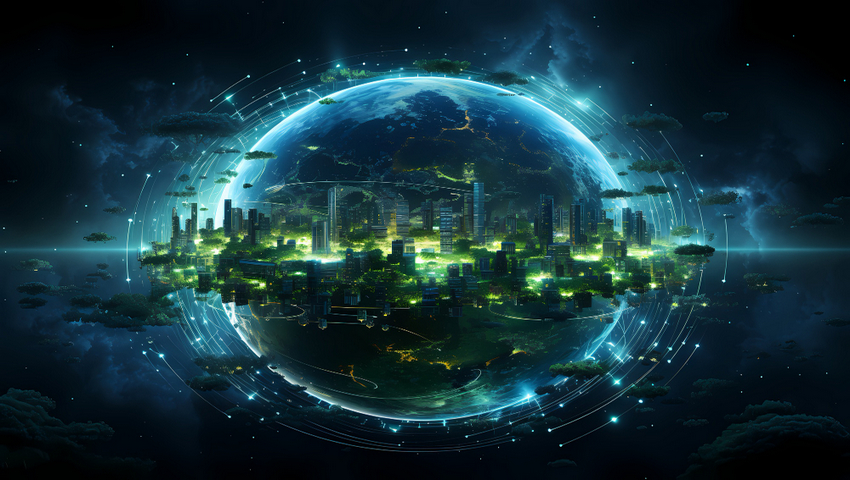
In the field of green energy, a powerful synergy takes place at the intersection of human intellect and technological innovation. Researchers from the University of Kyushu, the University of Osaka and the Fine Ceramic Center Blow a transformative course by integrating the capacities of automatic learning (ML) in the field of materials science. This collaboration not only accelerates the discovery of materials for green energy technology, but also contributes to new moments when artificial intelligence modifies the possibilities of scientific exploration.
The global quest for sustainable energy solutions has propelled scientists to explore unconventional paths. The solid oxide fuel cells, designed to generate energy from ecological fuels such as hydrogen, have become precursors in the race for carbon neutral sources of energy. However, conventional methodologies for the discovery of materials have posed significant challenges, limiting the scope of exploration. Recognize the transformer potential of AI researchers embarked on a mission to transcend these limits and redefine the landscape of materials science.
At the heart of this paradigm shift is a complete framework which transparently integrates calculation screening and high speed ML algorithms. This multidimensional approach allows researchers to dynamically explore materials beyond the constraints of traditional methods, releasing the full potential of AI in the pursuit of green energy.
In solid oxide fuel cells, the effective flow of hydrogen ions is essential for energy production. Here, ML appears as transformative forces. The research team takes advantage of automatic learning algorithms to analyze a wide range of oxides and dopants, deciphering the complex factors influencing the conductivity of protons. At the start of the traditional test and error methods, this AI approach to AI predicts optimal material combinations, accelerating speed and improving the accuracy of the discovery process.
The combination of AI and human intuition led to the rapid identification of two revolutionary materials for solid oxide fuel cells. A material, distinguished by its crystalline structure of Sillenite, marks the first conductive of known protons of the genre. Another material has a high -speed proton conduction path, which questions established standards. Although current conductivity levels are promising, researchers anticipate significant improvements thanks to more in -depth exploration.
The science of materials, with its complex challenges, finds a robust ally in AI and ML. Traditional approaches are often confronted with complexities resulting from punctual defects in materials. Enter the model learning models formed by defects chemistry, transparent this complex landscape. These models provide not only quantitative predictions, but also provide crucial information to select synthesizable host doping combinations, illustrating the ML transformer potential in the science of materials.
While we hold at the crossroads of the scientific survey and technological prowess, the fusion of AI propels us towards a future where green energy solutions are not only aspirations but tangible realities. Beyond the immediate progress of the discovery of materials, this collaboration establishes a precedent for the pivotal role that ML can play in the formation of the trajectory of scientific exploration. With each discovery, we get closer to a world where sustainable energy solutions are an integral part of our collective future, propelled by the unlimited potential of human-AI partnerships.
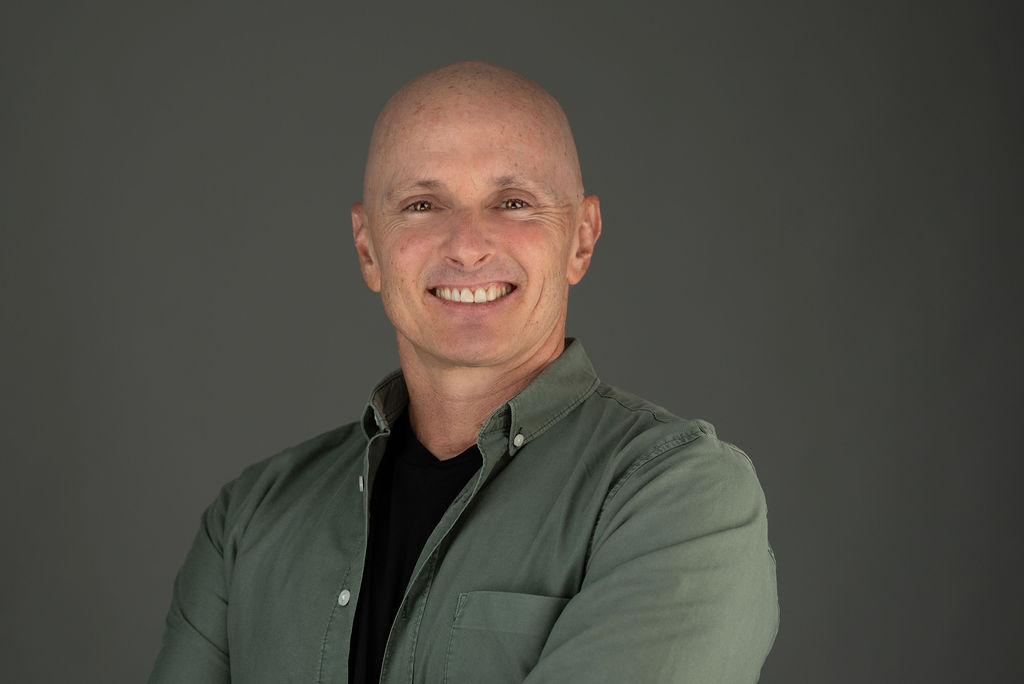