A domestic robot formed to perform household tasks in a factory may not effectively rub the sink or eliminate garbage when deployed in a user's kitchen, because this new environment differs from its training space.
To avoid this, engineers often try to match the simulated training environment as closely as possible with the real world where the agent will be deployed.
However, MIT researchers have now found that, despite this conventional wisdom, the training sometimes in a completely different environment gives a better efficient artificial intelligence agent.
Their results indicate that, in certain situations, the formation of an AI agent simulated in a world with less uncertainty, or “noise”, allowed him to perform better than a competitor agent formed in the same noisy world they used to test the two agents.
Researchers call for this unexpected phenomenon the indoor formation effect.
“If we learn to play tennis in an inner environment where there is no noise, we may be able to master different plans more easily. Then, if we go to a noisier environment, like a windy tennis court, we may have a higher probability of playing tennis well than if we are starting to learn in the windy environment, “explains Serena Bono, a research assistant in the MIT media laboratory and the main author of an article on the Audor training effect.
The interior training effect: unexpected gains of distribution changes in the transition function
Video: Mit Center for Brains, Minds and Machines
The researchers studied this phenomenon by forming AI agents to play atari games, which they modified by adding a certain unpredictability. They were surprised to note that the indoor training effect occurred through Atari games and variations in play.
They hope that these results will fuel additional research to develop better training methods for AI agents.
“This is an entirely new axis to think. Rather than trying to match training and testing environments, we can be able to build simulated environments where an AI agent learns even better, “adds co-author Spandan Madan, a student graduated at Harvard University.
Bono and Madan are joined on the newspaper by Ishaan Grover, a student graduated from MIT; Mao Yasueda, a graduate student at the University of Yale; Cynthia Breazeal, professor of arts and media sciences and leader of the personal robotics group in MIT Media Lab; Hanspeter Pfister, Professor An Wang of Computer Science at Harvard; And Gabriel Kreiman, professor at the Harvard Medical School. Research will be presented to the association for the Advancement of Artificial Intelligence Conference.
Training disorders
Researchers have decided to explore why strengthening learning agents tend to have such dismal performance when they are tested in environments that differ from their training space.
Learning strengthening is a test and error method in which the agent explores a training space and learns to take measures that maximize his reward.
The team has developed a technique to explicitly add a certain amount of noise to an element of the strengthening learning problem called the transition function. The transition function defines the probability that a agent goes from one state to another, depending on the action it chooses.
If the agent plays PAC-Man, a transition function could define the probability that the ghosts on the game board move up, the bottom, the left or the right. In learning standard strengthening, AI would be formed and tested using the same transition function.
The researchers added noise to the transition function with this conventional approach and, as expected, this has harmed the PAC-Man performance of the agent.
But when the researchers trained the agent with a noise-free Pac-Man game, then tested him in an environment where they injected noise into the transition function, he worked better than a ratings agent.
“The basic rule is that you should try to capture the transition function of the deployment condition as well as you can during the training to get the most out of it for your money. We really tested this idea to death because we could not believe it ourselves,” said Madan.
The injection of different quantities of noise in the transition function allows researchers to test many environments, but that has not created realistic games. The more noise in PAC-Man, the more the ghosts are likely to teleport to random on different squares.
To see if the indoor training effect occurred in the normal PAC-Man games, they adjusted the underlying probabilities so that the ghosts are moving normally but were more likely to go up and down, rather than on the left and on the right. AI agents formed in noise -free environments have worked even better in these realistic games.
“It was not only due to the way we added noise to create ad hoc environments. This seems to be a property of the problem of learning to strengthen. And it was even more surprising to see, ”explains Bono.
Exploration explanations
When the researchers dug more deeply in search of an explanation, they saw some correlations in the way in which the agents of the AI explore the training space.
When the two AI agents explore the same areas mainly, the agent trained in the non-numeal environment works better, perhaps because it is easier for the agent to learn the rules of the game without the interference of noise.
If their exploration models are different, the agent trained in the noisy environment tends to work better. This can happen because the agent must understand the models he cannot learn in the noise -free environment.
“If I only learn to play tennis with my forehand in the non -noise environment, but in the noisy, I also have to play with my setback, I will not play as well in the non -noise environment,” explains Bono.
In the future, researchers hope to explore how indoor training effect could occur in more complex strengthening learning environments, or with other techniques such as computer vision and natural language processing. They also wish to build training environments designed to take advantage of the interior training effect, which could help AI agents to perform better in uncertain environments.
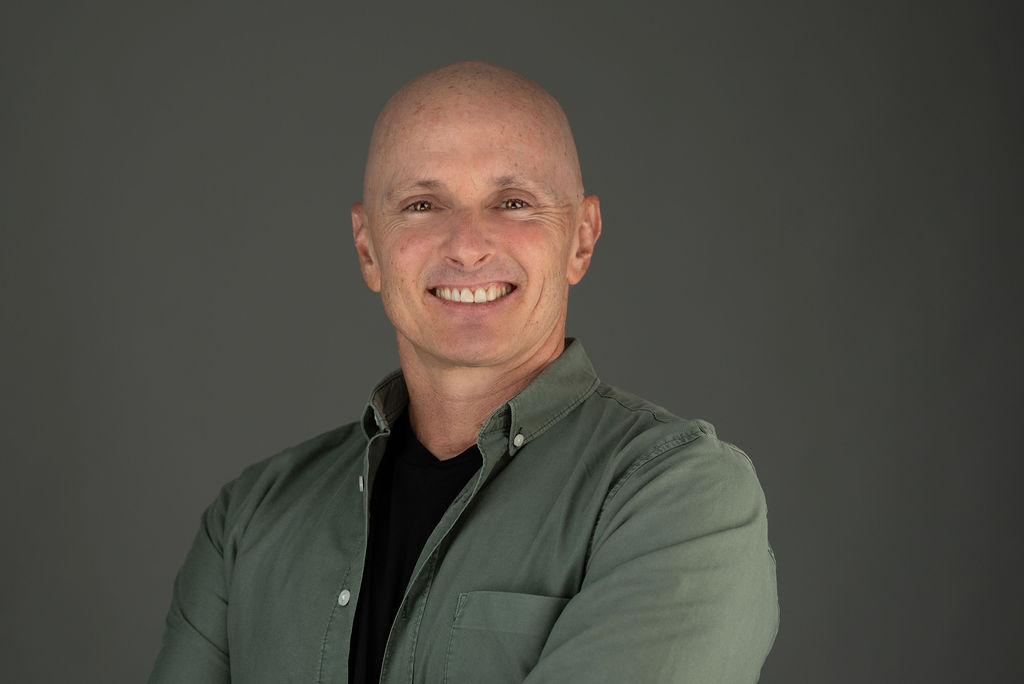