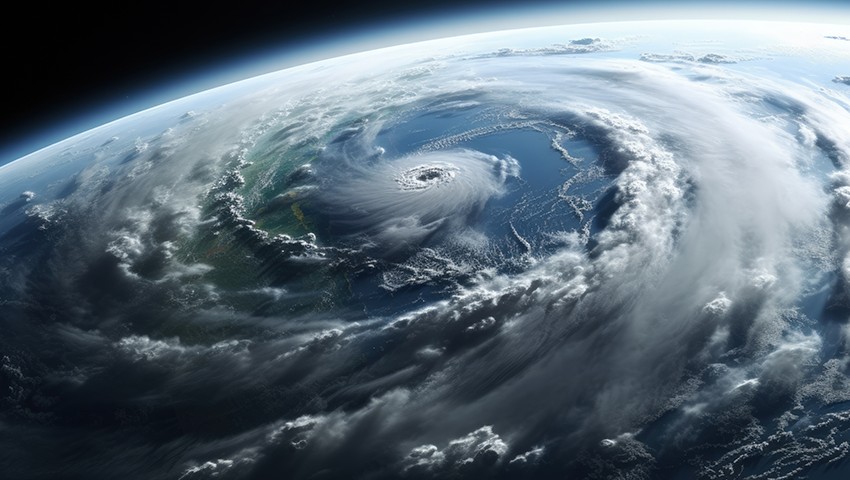
With the advent of artificial intelligence (AI) in weather forecasts, which once seemed to be an insurmountable challenge – predict the path and the intensity of hurricanes – now undergoes enormous transformation. Advanced AI models announce a paradigm shift in forecastingOffering unprecedented clarification and potential information on one of the most formidable phenomena of nature: the Hurricane of the Atlantic.
The journey to weather forecasts focused on AI started with a pivotal moment – a fortuitous meeting between two young innovators at the University of Stanford. John Dean, an electrician engineer, and Kai Marshland, a computer scientist, embarked on a mission to solve the secular problem of meteorological uncertainty. Their original and wind systems have introduced a new solution: miniature meteorological balloons, capable of crossing the earth's atmosphere for 40 days. By exploiting these balloons to collect atmospheric data, the dean and the marsh aimed to revolutionize global weather forecasts, transcending the limits of traditional observation methods.
Before that, the European Center for Middle Meeting Forecasts (ECMWF) has maintained a treasure of data called ERA5. This data set contains atmospheric, terrestrial and oceanic weather data for each day, to points from around the world, dating back to 1940. The last 50 years of data, in particular after the advent of global satellite coverage, are particularly rich. Although the ERA5 was not initially created for AI applications, it has proven incredibly useful for forming weather forecast models.
Computer scientists began to seriously use ERA5 data to train AI models for weather forecasts in 2022. Since then, technology has made rapid progress. In some cases, the production of these AI models exceeds global weather models that scientists have spent decades to design and build. And here is the botter: these AI models can work on a single desktop computer, unlike their supercomputer counterparts.
The transition from theoretical innovation to the application of the real world has been rapid. Windborne's Weathmemesh model, powered by AI algorithms, has demonstrated remarkable prowess in hurricanes forecasts, outperforming traditional models based on physics in the precision of the prediction of the track. Likewise, the ECMWF adopted the AI ​​revolution, revealing the artificial intelligence system / Integrated forecast (AIF). With its ability to produce “very promising” forecasts in real time, AIFs represent a significant jump forward in the weather prediction capacities.
At the heart of this meteorological, Marvel is a fundamental change in approach. Unlike traditional forecasting models, which are based on complex physical equations to simulate atmospheric dynamics, AI models like Weathemesh and AIFs work on a different principle – learning. By analyzing large data sets, including ERA5, these models discern complex models and correlations, allowing them to predict weather phenomena with unprecedented precision.
An instantaneous of the earth and its weather conditions is shown to the model: values ​​such as temperature, pressure, humidity, winds and much more at different levels of the atmosphere. Then the model is shown which conditions were like six hours later around the earth. The model “learns” this relationship between the weather now and conditions a few hours later. The process is repeated several times. By ingesting all this data, the model improves better and better to recognize the models and establish links on the conditions now, for example, a low pressure zone on the North Atlantic Ocean, and what it means for the weather downstream on Europe and Asia in the coming week at 10 days.
One of the most striking advantages of AI models is their effectiveness. Traditional models based on physics require enormous computing power, often relying on superordinators. On the other hand, AI models like Weathemesh can operate on a single powerful desktop computer equipped with a GPU. This spectacular reduction in calculation resources makes AI models more accessible and scalable.
While the meteorological community impatiently embraces the promise of forecasts focused on AI, the challenges abound. Ensure robustness, manipulation of extreme events and the fight against bias are critical areas of research. The integration of AI models into operational forecasts poses logistical and methodological obstacles, requiring rigorous evaluation and validation. In addition, the coexistence of models based on AI and based on physics raises questions about their additional roles in the formation of future forecasting methodologies.
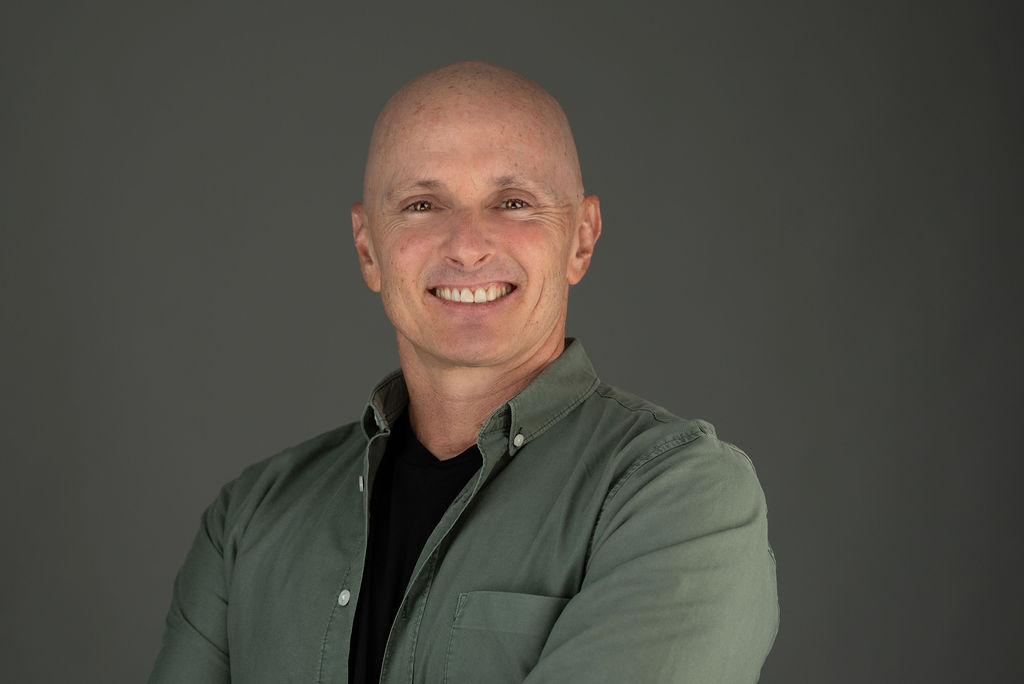