The self -regressive models (AR) have made significant progress in the generation of languages and are increasingly explored for image synthesis. However, the scaling of AR models to high resolution images remains a persistent challenge. Unlike the text, where relatively few tokens are necessary, high resolution images require thousands of tokens, which leads to quadratic growth in the calculation cost. Consequently, most multimodal models based on AR are limited to low or medium resolutions, limiting their usefulness for a generation of detailed images. Although the diffusion models have shown solid performance to high resolutions, they are delivered with their own limitations, including complex sampling procedures and slower inference. The fight against the bottleneck of the effectiveness of tokens in AR models remains an important open problem to allow a synthesis of high -resolution and practical image.
Meta Ai has token-shuffes
Meta Ai Present TokenA method designed to reduce the number of image tokens processed by transformers without modifying the fundamental prediction scope of the neighbor. The key computer computer which underlies token-shuffes is the recognition of dimensional redundancy in the visual vocabulars used by multimodal models of large language (MLLMS). Visual tokens, generally derived from vector quantification models (VQ), occupy large spaces but bear a lower intrinsic information density compared to text tokens. The token tokens exploit this by merging the spatially local visual tokens along the dimension of the canal before the treatment of the transformer and then in the restaurant of the original spatial structure after inference. This token merger mechanism allows AR models to manage higher resolutions with a considerably reduced calculation cost while maintaining visual fidelity.

Technical details and social benefits
Token-Shuffle consists of two operations: token And token-inhuff. During the preparation of the entries, spatially neighboring tokens are merged using an MLP to form a compressed token that preserves essential local information. For a Shuffle window size SSS, the number of tokens is reduced by a factor of S2S ^ 2S2, leading to a substantial reduction in transformer flops. After the transformer layers, the token-unhuffle operation reconstructs the original space layout, again helped by light MLP.
By compressing the token sequences during the transformer calculation, the cases of token allows the effective generation of high-resolution images, including those with resolution 2048 × 2048. Above all, this approach does not require modifications to the architecture of the transformer itself, nor introduces auxiliary loss functions or withdrawal of additional encoders.
In addition, the method incorporates a Consulting planner without classifier (CFG) Specifically suitable for autoregressive generation. Rather than applying a fixed guidance scale on all tokens, the planner gradually adjusts the guidance force, minimization of early tokens artefacts and improving the alignment of the image of the text.
Empirical results and ideas
The token-shuffes were evaluated on two major references: Genai-Bench And Awesome. On Genai-Bench, using a model based on a 2.7b parameter, token-shuffes have produced a VQASCORE of 0.77 on “hard” guestsoutperforming other self -regressive models such as Llamagen by a +0.18 margin and diffusion models like LDM of +0.15. In the Geneval reference, he reached a global score of 0.62Definition of a new reference base for the MAD models operating in the discreet tokens regime.
Large -scale human evaluation also supported these results. Compared to Llamagen, Lumina-Mgpt and the basic diffusion bases, Token-Shuffes have shown improved alignment with textual prompts, reduced visual defects and higher subjective image quality in most cases. However, a minor degradation of logical coherence was observed compared to the diffusion models, suggesting paths for additional refinement.
In terms of visual quality, token-shuffes have demonstrated the ability to produce detailed and coherent images 1024 × 1024 and 2048 × 2048. Ablation studies have revealed that smaller mixture window sizes (for example, 2 × 2) offered the best compromise between calculation efficiency and output quality. The larger window sizes have provided additional accelerated, but have introduced minor losses into fine grain details.

Conclusion
Token-shuffle presents a simple and effective method to meet the scalability limits of the generation of self-regressive images. By taking advantage of the redundancy inherent in visual vocabularies, it achieves substantial reductions in the calculation cost while preserving and, in some cases, improving the quality of production. The method remains entirely compatible with existing prediction frames, which facilitates integration into multimodal systems based on standard AR.
The results demonstrate that token-shuffes can push AR models beyond the previous resolution limits, which makes the generation high fidelity and high resolution more practical and more accessible. While research continues to advance the scalable multimodal generation, token-shuffes offer a promising base for effective unified models capable of managing large-scale text and image methods.
Discover the Paper. Also, don't forget to follow us Twitter And join our Telegram And Linkedin Group. Don't forget to join our 90K + ML Subdreddit.
Asif Razzaq is the CEO of Marktechpost Media Inc .. as a visionary entrepreneur and engineer, AIF undertakes to exploit the potential of artificial intelligence for social good. His most recent company is the launch of an artificial intelligence media platform, Marktechpost, which stands out from its in-depth coverage of automatic learning and in-depth learning news which are both technically solid and easily understandable by a large audience. The platform has more than 2 million monthly views, illustrating its popularity with the public.
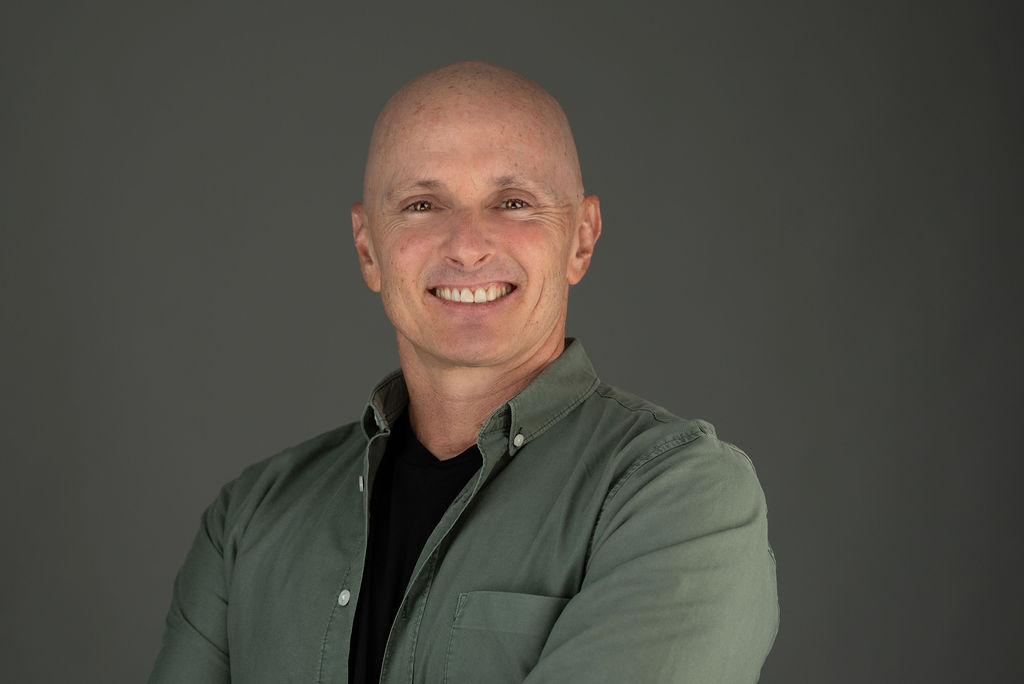