The design and evaluation of web interfaces are one of the most critical tasks in the current digital-premier world. Each modification of the provision, the positioning of the elements or the navigation logic can influence the way users interact with websites. It becomes even more crucial for platforms based on a thorough commitment of users, such as electronic commerce or content streaming services. One of the most reliable methods to assess the impact of design changes is A / B tests. In A / B tests, two or more versions of web page are shown to different user groups to measure their behavior and determine which variant works better. It is not only a question of aesthetics but also of functional usability. This method allows product teams to bring together evidence focused on the user before completely deploying functionality, allowing companies to systematically optimize user interfaces according to the interactions observed.
Although it is a widely accepted tool, the traditional A / B test process brings several ineffections that have proven to be problematic for many teams. The most important challenge is the volume of the real user traffic necessary to give statistically valid results. In some scenarios, hundreds of thousands of users must interact with web page variants to identify significant models. For smaller websites or features at an early stage, securing this level of user interaction may be almost impossible. The feedback cycle is also particularly slow. Even after having launched an experience, it can take weeks to months before the results can be evaluated with confidence due to the requirement of long observation periods. In addition, these tests are heavy with resources; Only a few variants can be assessed due to the required time and workforce. Therefore, many promising ideas are not tested because there is simply no ability to explore them all.
Several methods have been explored to overcome these limits; However, everyone has their shortcomings. For example, offline A / B test techniques depend on the rich historic interaction newspapers, which are not always available or reliable. The tools that allow prototyping and experimentation, such as appearance and fuse, have accelerated an early design exploration but are mainly useful for the prototyping of physical interfaces. The algorithms that reframe A / B tests as a research problem via evolutionary models help automate certain aspects but still depend on historical or real deployment data. Other strategies, such as cognitive modeling with GOMS or ACT-R frames, require high levels of manual configuration and do not easily adapt to dynamic web behavior complexities. These tools, although innovative, have not provided the scalability and automation necessary to respond to the deeper structural limitations of the A / B test workflows.
Researchers from the Northeastern University, Pennsylvania State University and Amazon presented a new automated system named Agenta / B. This system offers an alternative approach to traditional user tests, using Great language model (LLM) Based agents. Rather than depending on the interaction of live users, Agenta / B simulates human behavior using thousands of AI agents. These agents are assigned detailed characters who imitate characteristics such as age, school history, technical competence and purchasing preferences. These characters allow agents to simulate a wide range of user interactions on real websites. The objective is to provide researchers and product managers an effective and scalable method to test several design variants without counting on the comments of live users or the coordination of in -depth traffic.
Agea / B system architecture is structured in four main components. First of all, it generates agent characters based on entry demography and behavioral diversity specified by the user. These characters are introduced in the second step, where test scenarios are defined – this includes the allocation of agents to control and processing groups and specifying the two web pages versions must be tested. The third component performs interactions: agents are deployed in real browser environments, where they process content via structured web data (converted into JSON observations) and take measures as real users. They can search, filter, click and even simulate purchases. The fourth and last component is to analyze the results, where the system provides measures such as the number of clicks, purchases or interaction durations to assess the effectiveness of the design.
During their test phase, researchers used Amazon.com to demonstrate the practical value of the tool. In total, 100,000 virtual customer characters were generated and 1,000 were randomly selected from this pool to act as LLM agents in the simulation. The experience compared two different web pages provisions: one with all the product filter options indicated in a left panel and another with only a reduced set of filters. The result was convincing. The agents interacting with the reduced filter version made more purchases and actions based on filters than those with the full list. In addition, these virtual agents were much more effective. Compared to a million real user interactions, LLM agents have taken fewer actions on average to perform tasks, indicating more behavior focused on objectives. These results reflected the behavioral direction observed in human A / B tests, strengthening the case for the agea / b in supplement valid to traditional tests.
This research demonstrates convincing progress in the assessment of the interface. It is not intended to replace the A / B tests of the user live, but rather offers an additional method which offers rapid feedback, a larger profitability and experimental coverage. Using AI agents instead of live participants, the system allows teams to test many interface variations which would be otherwise impracticable. This model can considerably compress the design cycle, allowing ideas to be validated or rejected at a much earlier stage. It responds to the practical concerns of long waiting times, traffic limitations and resource constraints, making the web design process more focused on data and less subject to bottlenecks.
Some key dishes in agea / b research include:
- Agenta / B uses LLM -based agents to simulate the realistic behavior of users on live web pages.
- The system allows automated A / B tests without the need to deploy live users.
- 100,000 user characters have been generated and 1,000 were selected for the simulation of live tests.
- The system compared two web page variants on Amazon.com: full filter panel compared to reduced filters.
- LLM agents in the reduced filter group made more purchases and made more filtering actions.
- Compared to 1 million human users, LLM agents have shown shorter action of action and more behavior led by objectives.
- Agenta / B can help assess interface changes before user real tests, saves months of development time.
- The system is modular and expandable, which allows it to be adaptable to various web platforms and to test objectives.
- It responds directly to three basic A / B test challenges: long cycles, high needs of user traffic and experience failure rates.
Discover the Paper. Also, don't forget to follow us Twitter And join our Telegram And Linkedin Group. Don't forget to join our 90K + ML Subdreddit.
Sana Hassan, consulting trainee at Marktechpost and double -degree student at Iit Madras, is passionate about the application of technology and AI to meet the challenges of the real world. With a great interest in solving practical problems, it brings a new perspective to the intersection of AI and real life solutions.
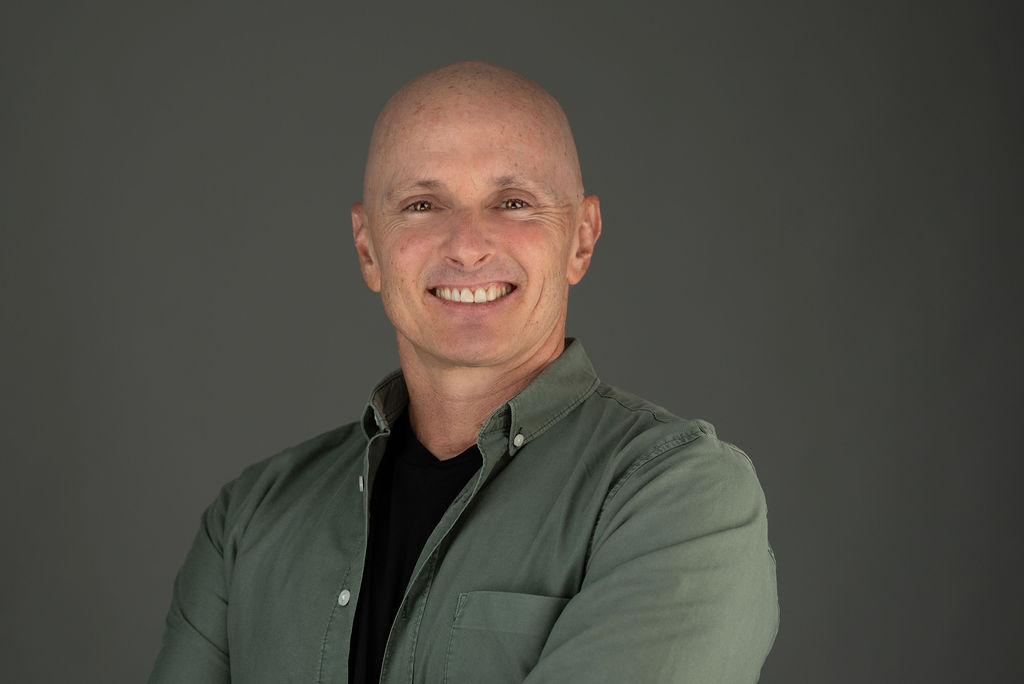